Unleashing the Potential of Large Language Models in AI
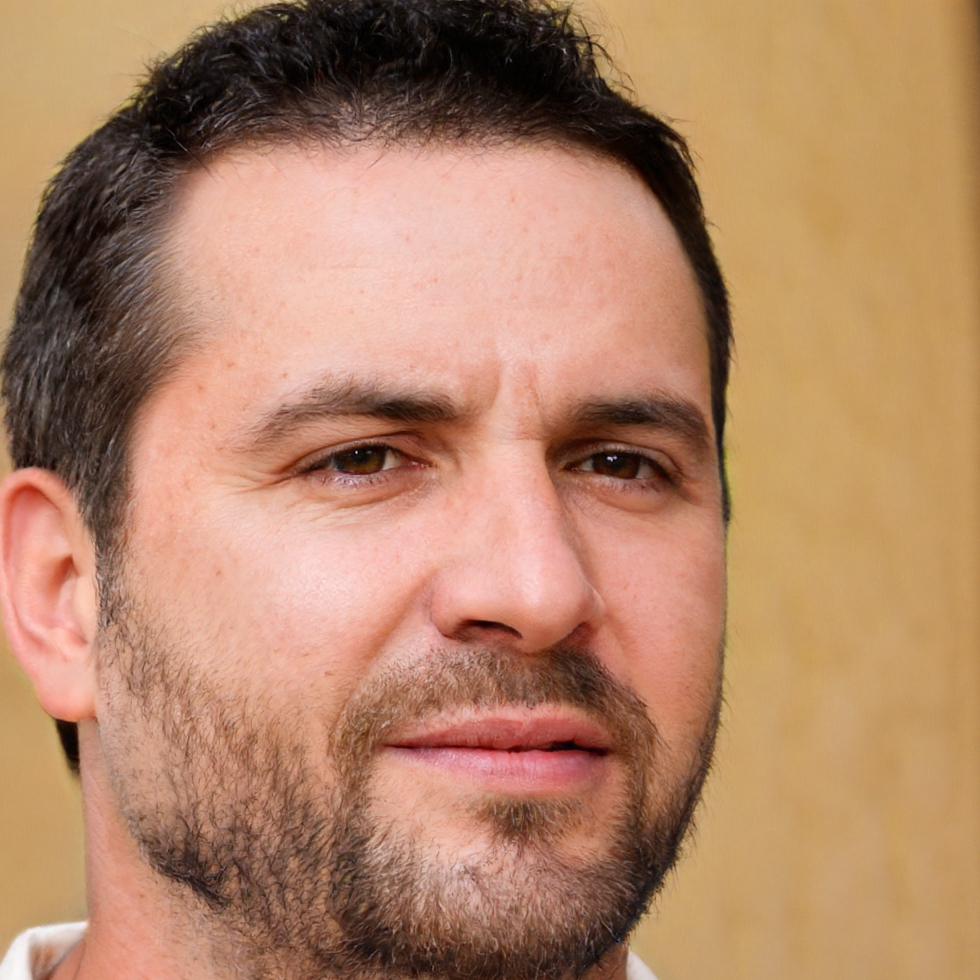
Author
Oliver ThompsonUnleashing the Potential of Large Language Models in AI explores the advancements, challenges, and ethical considerations surrounding the use of large language models in artificial intelligence. This article delves into the understanding of these models, their applications, and the latest advancements in the field. Ultimately, it aims to shed light on how these models can revolutionize the way we interact with AI technologies.
Introduction
In recent years, large language models have become a significant focus in the field of Artificial Intelligence (AI). These models, powered by deep learning techniques, have shown remarkable advancements in various natural language processing tasks, such as language generation, translation, summarization, and more. The ability of these models to understand, generate, and manipulate human language has opened up a wide range of applications in industries such as technology, healthcare, finance, and media.
The rise of large language models can be attributed to the availability of vast amounts of text data, powerful computational resources, and innovative algorithms. Companies like OpenAI, Google, and Facebook have made significant investments in developing and scaling these models to unprecedented sizes, such as GPT-3 with 175 billion parameters.
Despite their impressive capabilities, large language models also pose several challenges and ethical considerations. The sheer size and complexity of these models make training and fine-tuning them a daunting task. Additionally, concerns have been raised about the bias and security risks associated with deploying these models in real-world scenarios.
In this article, we will explore the underlying principles of large language models, the challenges in training and deploying them, the diverse applications they can be used for, and the ethical implications that come with their development. We will also discuss the latest advancements in large language models and their potential to revolutionize the field of artificial intelligence.
Understanding Large Language Models
Large Language Models (LLMs) are a type of artificial intelligence (AI) model that has gained significant attention in recent years due to their ability to generate human-like text. These models are typically trained on massive amounts of textual data, such as books, articles, and online resources, in order to learn the patterns and structure of language.
At the core of LLMs is a deep neural network that processes input text and generates output text based on the patterns it has learned during training. The network is typically trained using a technique called unsupervised learning, where the model learns to predict the next word in a sequence of text based on the words that came before it.
One of the key features of LLMs is their ability to generate coherent and contextually relevant text. This is achieved through a technique called self-attention, which allows the model to weigh the importance of different words in a sentence based on their context. By doing so, the model can generate text that is not only grammatically correct but also coherent and contextually appropriate.
Another important aspect of LLMs is their ability to generate text that is diverse and creative. This is achieved through a technique called sampling, where the model generates multiple possible outputs and selects the most relevant or interesting one. This allows LLMs to generate text that is not only correct but also interesting and engaging to read.
In addition to text generation, LLMs can also be used for a variety of other tasks, such as translation, summarization, and question answering. These models are often fine-tuned on specific tasks to improve their performance and accuracy.
However, despite their impressive capabilities, LLMs also come with several challenges. One of the main challenges is the need for massive amounts of computational resources and data to train these models effectively. Additionally, there are concerns about the ethical implications of using LLMs, such as the potential for bias in the generated text.
In conclusion, understanding LLMs is crucial for unlocking their full potential in AI. By harnessing the power of these models, we can revolutionize how we interact with and use language in a wide range of applications.
Challenges in Training Large Language Models
Training large language models presents several challenges that need to be addressed in order to achieve optimal performance. These challenges can arise from various aspects of the training process, such as data preprocessing, model architecture, hyperparameters tuning, and computational resources.
Data Preprocessing Challenges
One of the primary challenges in training large language models is data preprocessing. Preprocessing involves cleaning and formatting the input data to make it suitable for training. This process can be time-consuming and resource-intensive, especially when dealing with large datasets. Furthermore, preprocessing text data can be challenging due to noise, inconsistencies, and linguistic variations in the data.
Model Architecture Challenges
Another challenge in training large language models is designing an effective model architecture. The architecture of a language model determines its ability to learn and generalize from the input data. Designing a scalable and efficient model architecture that can handle large amounts of data while maintaining high performance is a challenging task. Additionally, adapting existing architectures or creating new ones to suit specific use cases or applications can be a complex process.
Hyperparameters Tuning Challenges
Hyperparameters tuning is another critical aspect of training large language models. Hyperparameters, such as learning rate, batch size, and optimizer settings, play a crucial role in determining the performance of a model. Tuning these hyperparameters to achieve optimal performance can be challenging, as it often requires iterative experimentation and fine-tuning. Moreover, finding the right set of hyperparameters that balance training speed and model accuracy can be time-consuming and resource-intensive.
Computational Resources Challenges
Training large language models requires significant computational resources to handle the massive amounts of data and complex model architectures involved. High-performance computing resources, such as GPU clusters or TPUs, are often necessary to accelerate the training process and achieve satisfactory performance. However, accessing and managing these resources can be costly and logistically challenging, especially for research teams or organizations with limited budgets or infrastructure.
In conclusion, the challenges involved in training large language models are multifaceted and require careful consideration and strategic planning to overcome. Addressing these challenges effectively is crucial for unlocking the full potential of large language models in AI applications.
Applications of Large Language Models
Large language models have been proven to be incredibly versatile and have a wide range of applications across various industries. The capabilities of these models have enabled advancements in natural language processing and machine learning, leading to new and exciting opportunities for businesses and researchers alike.
1 Conversational Agents
One of the most popular applications of large language models is in the development of conversational agents. These agents, also known as chatbots or virtual assistants, use the power of large language models to understand, process, and generate human-like responses in natural language. Conversational agents are used in customer service, online support systems, and even in personal assistants like Siri and Google Assistant.
2 Text Generation
Large language models are also widely used for text generation tasks such as summarization, translation, and content creation. These models can generate coherent and contextually relevant text, making them valuable tools for writers, journalists, and content creators. Companies are utilizing large language models to automate content generation, improve SEO, and enhance user experience.
3 Sentiment Analysis
Another important application of large language models is in sentiment analysis. These models can analyze and interpret the emotions and opinions expressed in text data, helping businesses understand customer feedback, social media trends, and market sentiments. Sentiment analysis powered by large language models is used in social listening tools, customer satisfaction surveys, and brand reputation management.
4 Healthcare
Large language models are also making significant strides in the healthcare industry. Researchers are using these models for clinical decision support, medical image analysis, and drug discovery. Large language models can process vast amounts of medical literature and patient data to provide insights for diagnosis, treatment planning, and personalized medicine.
5 Financial Services
In the financial services sector, large language models are used for risk assessment, fraud detection, and investment analysis. These models can analyze text data from financial reports, market trends, and regulatory documents to provide real-time insights for decision-making. Large language models are also used for automated trading, credit scoring, and customer support in the banking and finance industry.
6 Education
Large language models are transforming the education sector by personalizing learning experiences, creating interactive content, and assessing student performance. These models can generate adaptive learning pathways, provide instant feedback to students, and assist teachers in creating engaging educational materials. Large language models are used in virtual classrooms, online tutoring, and educational chatbots to enhance the learning process.
Overall, the applications of large language models are vast and diverse, offering innovative solutions across various domains. As these models continue to evolve and improve, the potential for new and exciting applications in AI is limitless. Businesses and researchers are constantly exploring new ways to leverage the power of large language models to drive advancements in technology and improve human experiences.
Ethical Considerations in Large Language Models
Large language models have the potential to revolutionize various industries and improve many aspects of our lives. However, along with their benefits, ethical considerations have become a significant concern in the development and deployment of these models. As these models become more powerful and pervasive, it is essential to address and mitigate potential ethical issues.
1 Bias and Fairness
One of the primary ethical concerns surrounding large language models is bias. These models learn from vast amounts of data, which can include biases present in the training data. This can result in the perpetuation and amplification of existing biases, leading to unfair outcomes. For example, if a model is trained on data that is biased against a particular group, the model may produce biased or discriminatory results. It is crucial for developers and researchers to carefully consider and address biases in the training data to ensure fairness and equity in the output of these models.
2 Privacy and Security
Another important ethical consideration in large language models is privacy and security. These models often require access to large amounts of data to operate effectively. This raises concerns about the privacy of the data being used and the potential for misuse or unauthorized access. There is a risk that sensitive information could be exposed or exploited, leading to privacy breaches or security vulnerabilities. It is essential for organizations to implement robust security measures and adhere to strict privacy regulations to protect user data and ensure the integrity of these models.
3 Accountability and Transparency
The accountability and transparency of large language models are also critical ethical considerations. As these models become more complex and powerful, it can be challenging to understand how they arrive at their decisions. This lack of transparency can make it difficult to hold developers accountable for the outcomes produced by these models. It is essential for organizations to be transparent about how these models are designed, trained, and deployed. Additionally, mechanisms should be put in place to enable users to understand and challenge the decisions made by these models.
4 Misinformation and Manipulation
Large language models have the potential to generate vast amounts of content, including text, images, and videos. While this can be beneficial in many ways, it also raises concerns about the spread of misinformation and manipulation. These models could be used to generate fake news, misinformation, or propaganda, influencing public opinion and shaping narratives. It is crucial for organizations to implement measures to detect and combat misinformation produced by large language models, ensuring the integrity of information shared online.
5 Discrimination and Harm
The potential for discrimination and harm is another ethical consideration in large language models. These models have the power to influence decision-making processes in various domains, such as hiring, lending, and law enforcement. If not properly monitored and regulated, these models could perpetuate discrimination against certain groups or individuals, leading to harmful and unjust outcomes. Organizations must proactively address these issues and put safeguards in place to prevent discrimination and protect vulnerable populations.
In conclusion, while large language models hold tremendous potential for innovation and advancement, it is crucial to address ethical considerations to ensure that these models are developed and deployed responsibly. By addressing issues such as bias, privacy, transparency, misinformation, discrimination, and harm, we can harness the power of these models for the benefit of society while minimizing potential risks and negative impacts.
Advancements in Large Language Models
In recent years, there have been significant advancements in the field of large language models that have revolutionized the way we approach natural language processing tasks. These advancements have been made possible by breakthroughs in deep learning, natural language understanding, and computational power. In this section, we will explore some of the key advancements that have shaped the landscape of large language models.
Pretraining Techniques
One of the major advancements in large language models has been the development of more sophisticated pretraining techniques. Models such as BERT (Bidirectional Encoder Representations from Transformers) have demonstrated the power of pretraining on large text datasets to improve model performance on downstream tasks. By pretraining a model on a diverse range of text data, researchers have been able to create models that have a better understanding of language and can perform a wide range of natural language processing tasks with greater accuracy.
Scaling Models
Another area of advancement in large language models has been the scaling of models. Researchers have been able to train larger and more complex models by leveraging distributed computing techniques and improved hardware. By increasing the size of models, researchers have been able to achieve state-of-the-art results on a wide range of natural language processing tasks, including language modeling, text generation, and question answering.
Transfer Learning
Transfer learning has also been a key advancement in large language models. By fine-tuning pre-trained models on specific tasks or domains, researchers have been able to achieve impressive results with less data and computational resources. Transfer learning allows models to leverage knowledge learned from one task to improve performance on another task, making it a valuable technique for training large language models.
Multimodal Models
In recent years, there has been a growing interest in multimodal models that can process both text and other modalities such as images, audio, and video. By incorporating multiple modalities into a single model, researchers have been able to create more contextual and comprehensive representations of language. Multimodal models have shown promise in a variety of applications, including image captioning, video understanding, and speech recognition.
Self-Supervised Learning
Self-supervised learning has emerged as a powerful technique for training large language models without the need for labeled data. By framing natural language processing tasks as unsupervised learning tasks, researchers have been able to train models on vast amounts of text data without the need for human annotations. Self-supervised learning has proven to be an effective way to learn rich and generalizable representations of language.
Future Directions
As large language models continue to advance, researchers are exploring new directions to further improve model performance and capabilities. Some of the areas that researchers are currently exploring include few-shot learning, multilingual models, interpretability, and fairness. By addressing these challenges, researchers hope to create large language models that are more robust, efficient, and ethical.
In conclusion, the advancements in large language models have enabled breakthroughs in natural language processing and have the potential to revolutionize the way we interact with and understand language. By pushing the boundaries of what is possible with large language models, researchers are paving the way for a new era of AI innovation.