The Role of Large Language Models in Advancing Artificial Intelligence
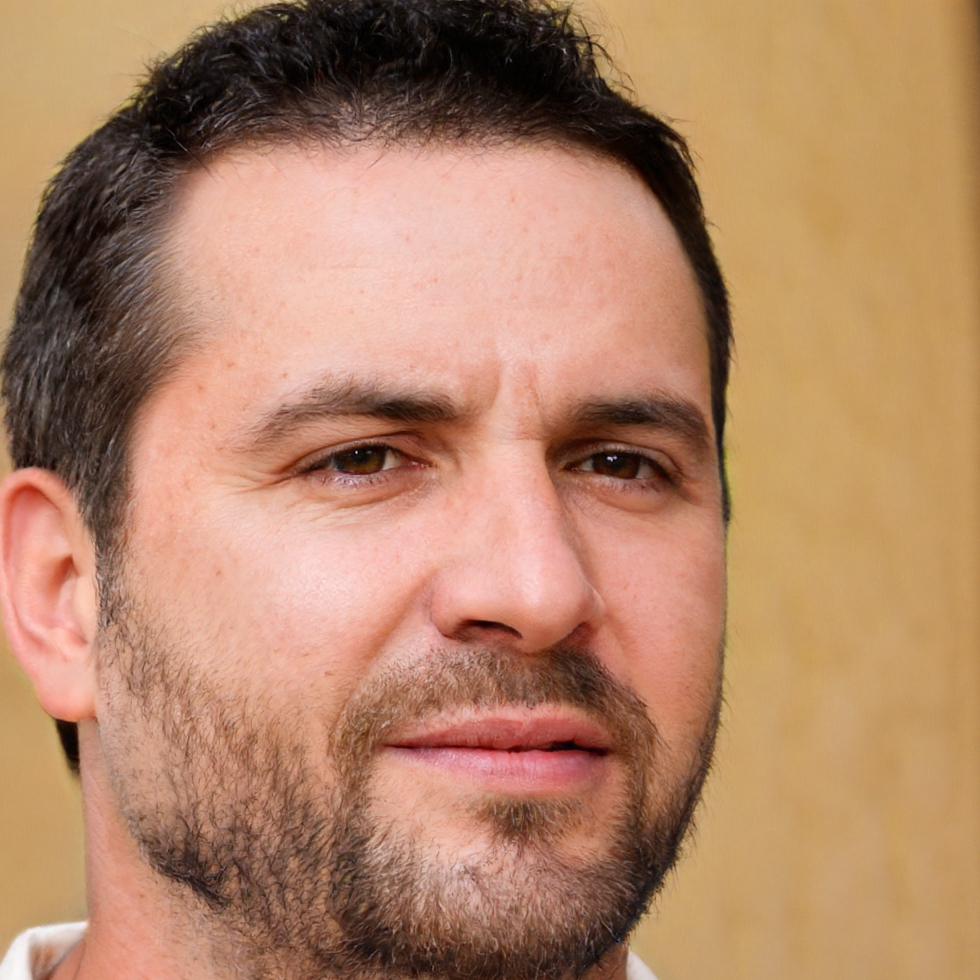
Author
Oliver ThompsonLarge language models have been at the forefront of advancing artificial intelligence in recent years, showcasing their potential in various applications. This article delves into the role of these models in shaping the future of AI, exploring their definition, key components, applications, as well as the challenges and limitations they present. Additionally, it provides insights into the ethical concerns and bias issues surrounding large language models, while also looking ahead at potential future directions in the field.
Introduction
In recent years, Large Language Models (LLMs) have emerged as a powerful tool in advancing Artificial Intelligence (AI) capabilities. These models, fueled by advancements in Deep Learning and Natural Language Processing (NLP), have demonstrated remarkable proficiency in a wide range of language-related tasks, from text generation to natural language understanding.
The ability of LLMs to process and generate human-like text has not only revolutionized the field of AI but has also raised significant ethical and societal concerns. As these models continue to evolve and grow in size and complexity, it becomes crucial to understand their inner workings, applications, challenges, and potential future directions.
This article aims to provide a comprehensive overview of the role of Large Language Models in advancing Artificial Intelligence. We will delve into the definition and history of LLMs, explore their key components, discuss their applications in natural language understanding, text generation, and dialogue systems, examine the challenges and limitations they face, such as ethical concerns and bias in language models, and speculate about the future directions of this rapidly evolving field.
By gaining a deeper understanding of LLMs and their impact on AI, we can better navigate the opportunities and challenges that lie ahead in the quest for smarter, more capable artificial intelligence systems.
Understanding Large Language Models
Large Language Models (LLMs) have become a significant area of research in the field of Artificial Intelligence (AI) due to their ability to process and generate human-like text. These models have revolutionized the way we interact with computers and have the potential to significantly impact various industries. To fully grasp the importance of LLMs, it is essential to understand their definition, history, and key components.
Definition and History
Large Language Models are deep learning algorithms trained on vast amounts of text data to understand and generate human language. These models are typically based on neural networks and use techniques like transformers to process text efficiently. One of the key advancements in the field of LLMs was the development of the GPT (Generative Pre-trained Transformer) series by OpenAI, which paved the way for more advanced models like GPT-3.
The history of LLMs can be traced back to early language models like n-gram models and Hidden Markov Models (HMMs). These models laid the foundation for the development of more complex neural network-based LLMs that can leverage large datasets and sophisticated architectures to achieve remarkable results.
Key Components of Large Language Models
Large Language Models consist of several key components that work together to process and generate human language. These components include:
Tokenization: The process of breaking down text into smaller units called tokens, which are then used as input to the model. Embeddings: Representations of words or tokens in a high-dimensional space, capturing their semantic and syntactic relationships. Encoder-Decoder Architecture: A common architecture used in LLMs, where an encoder processes input text and a decoder generates the output text. Attention Mechanism: A mechanism that allows the model to focus on relevant parts of the input text when generating output. Transformer Architecture: A type of neural network architecture that has shown great success in processing sequential data like text.
By understanding these key components, researchers and developers can design more efficient and effective LLMs that can tackle a wide range of natural language processing tasks.
In conclusion, Understanding Large Language Models is crucial for advancing the field of AI and unlocking the full potential of these powerful tools. By studying their definition, history, and key components, we can harness the power of LLMs to drive innovation and create new opportunities in the world of artificial intelligence.
Applications of Large Language Models
Large language models have a wide range of applications in various fields due to their ability to understand and generate human language. These models have shown significant advancements in Natural Language Understanding, Text Generation, and Dialogue Systems.
Natural Language Understanding
Large language models are being used in Natural Language Understanding tasks such as sentiment analysis, named entity recognition, and text classification. These models can extract meaning from text data and understand the context in which words are used. By training on vast amounts of text data, they can recognize patterns and nuances in language to improve their accuracy in understanding human language.
Text Generation
Large language models are also capable of generating human-like text. They can be used in Text Generation tasks such as content creation, automated storytelling, and language translation. These models can produce coherent and contextually relevant text by predicting the next word in a sentence based on the previous words. They have been employed in various applications, including chatbots, virtual assistants, and content creation tools.
Dialogue Systems
Large language models are crucial for Dialogue Systems, which aim to create interactive conversations between humans and machines. These systems use natural language processing techniques to understand and respond to user inputs in a conversational manner. By leveraging large language models, these systems can provide more engaging and personalized interactions with users, leading to improved user experience and satisfaction.
Challenges and Limitations
Large language models have shown tremendous potential in advancing artificial intelligence, but they also come with challenges and limitations that need to be addressed. These challenges range from ethical concerns to issues of bias in language models. In this section, we will explore some of the key challenges and limitations facing large language models.
Ethical Concerns
One of the most pressing challenges surrounding large language models is the ethical concerns that arise from their use. These models have the ability to generate highly convincing fake text, which can be used to spread misinformation or manipulate public opinion. This raises questions about the responsibility of researchers and developers in ensuring that these models are used ethically and responsibly.
Another ethical concern is the potential for large language models to perpetuate existing biases and stereotypes. If the data used to train these models is biased, the models themselves may exhibit bias in their output. This can have serious implications for decision-making processes in areas such as hiring, lending, and criminal justice.
Bias in Language Models
Bias in language models is a major limitation that can have far-reaching consequences. One of the main sources of bias in these models is the data used to train them. If the training data is not representative of the full range of human experiences and perspectives, the model will reflect and amplify these biases in its output.
Bias in language models can manifest in various ways, including gender bias, racial bias, and cultural bias. For example, a language model trained on a dataset that is predominantly male-dominated may generate text that reflects and perpetuates gender stereotypes. Similarly, a model trained on text from a specific cultural group may struggle to accurately capture the nuances of language from other cultural backgrounds.
Addressing bias in language models is a complex and ongoing challenge that requires a multi-faceted approach. Researchers and developers must carefully curate training data, implement bias mitigation techniques, and engage with diverse communities to ensure that these models are as fair and inclusive as possible.
In conclusion, while large language models hold great promise for advancing artificial intelligence, it is essential to acknowledge and address the challenges and limitations they present. By proactively addressing issues such as ethical concerns and bias, we can harness the full potential of these models while mitigating their negative impacts on society.
Future Directions
As large language models continue to advance and evolve, there are several key areas where future research and development could lead to significant progress in the field of artificial intelligence. These future directions encompass a wide range of possibilities, from improving model performance to addressing ethical concerns and biases. Below are some of the potential future directions for large language models:
Enhanced Performance
One of the most important future directions for large language models is improving their performance. This can involve increasing the size of the models, refining the training data, and enhancing the algorithms used to train them. By enhancing performance, language models can become even more accurate and capable of handling a wider range of tasks with greater efficiency.
Multi-Modal Models
Another promising future direction is the development of multi-modal models that can process and understand both text and other forms of data, such as images and videos. By combining multiple modalities, language models can gain a deeper understanding of the world and better interpret complex information. This could open up new possibilities for natural language processing and artificial intelligence applications.
Personalized Models
Personalized language models that are tailored to individual users could also be a key future direction. By personalizing models, language models can adapt to the specific needs and preferences of each user, providing more relevant and accurate responses. This could lead to a more customized and effective user experience in various applications.
Ethical AI
Addressing ethical concerns related to large language models is a critical future direction. Ensuring that language models are used responsibly and ethically, avoiding harmful biases and protecting user privacy, is essential for the long-term success and acceptance of AI technologies. Ethical guidelines and regulations must be developed and implemented to govern the use of language models in a fair and transparent manner.
Interpretability and Explainability
Improving the interpretability and explainability of language models is another important future direction. By making models more transparent and understandable, researchers can gain insights into how models make decisions and improve their trustworthiness. This could help to identify and address biases, errors, and limitations in language models, enhancing their overall reliability and utility.
Collaboration and Open-Source Initiatives
Collaboration among researchers and open-source initiatives are crucial future directions for advancing large language models. By sharing data, resources, and models in an open and collaborative manner, the AI community can accelerate innovation and progress in the field. Open-source projects enable researchers to build on each other's work, fostering knowledge sharing and cooperation.
In conclusion, the future directions for large language models are diverse and exciting, with numerous opportunities for advancements in artificial intelligence. By continuing to explore new ideas and technologies, researchers can push the boundaries of language understanding and AI capabilities, ultimately leading to more powerful and beneficial applications of large language models.