The Role of Deep Learning in Autonomous Vehicles and AI
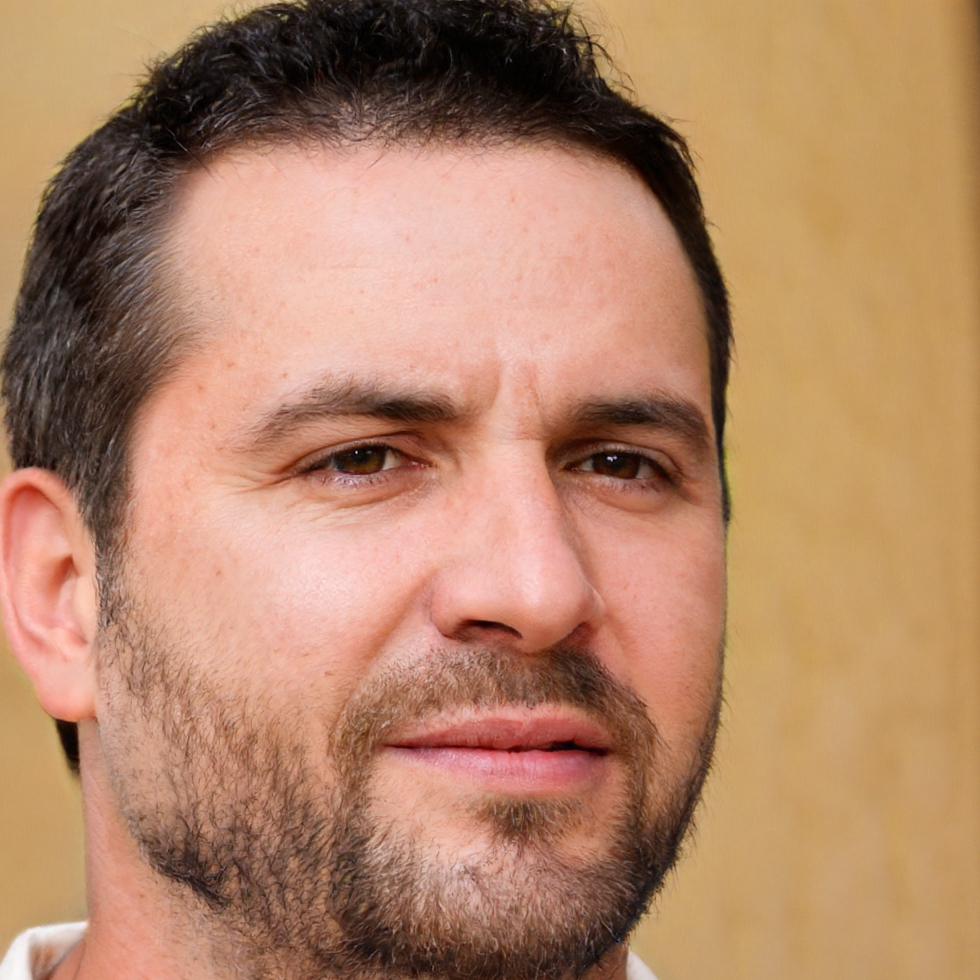
Author
Oliver ThompsonThis article explores the role of Deep Learning in Autonomous Vehicles and AI, discussing the evolution of autonomous vehicles, the principles of deep learning in this field, various applications, as well as challenges and limitations. Additionally, a look into the future trends of autonomous vehicles and AI is also provided.
Introduction
Autonomous vehicles, also known as self-driving cars, have been the subject of intense research and development in recent years. These vehicles have the potential to revolutionize the way we travel and could have a significant impact on safety, efficiency, and environmental sustainability. One of the key technologies driving the development of autonomous vehicles is deep learning, a type of artificial intelligence (AI) that is inspired by the structure and function of the human brain.
Deep learning has revolutionized many fields, including computer vision, natural language processing, and speech recognition. In the context of autonomous vehicles, deep learning plays a crucial role in enabling the vehicle to perceive its environment, make decisions, and navigate safely. By analyzing large amounts of data from sensors such as cameras, radar, and lidar, deep learning algorithms can learn to recognize objects, predict their movements, and react accordingly.
This article will explore the role of deep learning in autonomous vehicles, discussing the evolution of autonomous vehicles, the fundamentals of deep learning, the applications of deep learning in autonomous vehicles, the challenges and limitations of the technology, and future trends in the field. By the end of this article, readers will have a comprehensive understanding of the current state of autonomous vehicle technology and the potential for future advancements.
The Evolution of Autonomous Vehicles
Autonomous vehicles have come a long way since their inception, with significant advancements being made in recent years. The evolution of autonomous vehicles can be traced back to the early 20th century, when the idea of self-driving cars first emerged. However, it wasn't until the late 20th century and early 21st century that technology began to catch up with this ambitious vision.
One of the earliest examples of autonomous vehicles can be seen in the form of "Stanley," a modified Volkswagen Touareg that won the 2005 DARPA Grand Challenge. This event marked a significant turning point in the development of autonomous vehicles, demonstrating the capabilities of machine learning and artificial intelligence in creating vehicles capable of navigating complex terrains without human intervention.
Over the years, major automobile companies such as Tesla, Google's Waymo, and Uber have invested heavily in the research and development of autonomous vehicles. These companies have made significant strides in the field, with Tesla's Autopilot system and Waymo's self-driving vehicles being among the most well-known examples of autonomous technology in action.
The evolution of autonomous vehicles has been driven by a combination of technological advancements and regulatory changes. As sensors and computing power have become more sophisticated and affordable, autonomous vehicles have become increasingly capable of navigating real-world environments. Additionally, changes in legislation and public perception have paved the way for the widespread adoption of autonomous vehicles on public roads.
Looking ahead, the future of autonomous vehicles is promising, with predictions suggesting that self-driving cars will become mainstream within the next decade. As deep learning algorithms continue to improve and data sets become more abundant, autonomous vehicles will become even safer, more efficient, and more accessible to the general public. The evolution of autonomous vehicles is set to revolutionize the way we think about transportation, offering numerous benefits in terms of safety, efficiency, and sustainability.
Deep Learning in Autonomous Vehicles
Deep learning plays a crucial role in the development of autonomous vehicles by enabling them to perceive and understand the environment around them. It is a subset of machine learning that uses neural networks to mimic human brain functions and process large amounts of data to make decisions.
1 How Does Deep Learning Work in Autonomous Vehicles?
In autonomous vehicles, deep learning algorithms are trained using data collected from various sensors such as cameras, LiDAR, radar, and GPS. These algorithms analyze the data to recognize objects on the road, such as pedestrians, cyclists, other vehicles, and road signs. By continuously learning from the data, the deep learning models improve their accuracy and performance over time.
2 Object Detection and Recognition
One of the key tasks of deep learning in autonomous vehicles is object detection and recognition. Deep learning models can accurately identify and classify objects in real-time, allowing the vehicle to react accordingly. For example, if a pedestrian suddenly crosses the road, the vehicle can detect the person and brake to avoid a collision.
3 Semantic Segmentation
Semantic segmentation is another important application of deep learning in autonomous vehicles. It involves dividing an image into distinct regions or segments and assigning a label to each segment. This allows the vehicle to understand the context of the environment and make informed decisions. For example, semantic segmentation can help the vehicle differentiate between lanes, sidewalks, and buildings on the road.
4 Path Planning and Prediction
Deep learning also plays a crucial role in path planning and prediction for autonomous vehicles. By analyzing historical data and real-time inputs, deep learning models can predict the path of other vehicles on the road and plan the optimal route for the autonomous vehicle. This helps in avoiding obstacles and ensuring a safe journey.
5 Decision Making
In autonomous vehicles, deep learning algorithms are responsible for making real-time decisions based on the input data. These decisions include speed control, lane changing, turning, and stopping. By continuously analyzing the environment and predicting the actions of other road users, the vehicle can make safe and efficient decisions to reach its destination.
6 Continuous Learning and Adaptation
One of the key advantages of deep learning in autonomous vehicles is its ability to continuously learn and adapt to changing road conditions. As the vehicle encounters new situations and challenges, the deep learning models can update their knowledge and improve their performance. This ensures that the vehicle remains reliable and safe in various driving scenarios.
Overall, deep learning plays a vital role in the development of autonomous vehicles by enabling them to perceive, understand, and navigate the complex road environment. As deep learning algorithms continue to evolve and improve, we can expect to see safer, more efficient, and more autonomous vehicles on the roads in the near future.
Applications of Deep Learning in Autonomous Vehicles
Deep learning plays a crucial role in the development and implementation of autonomous vehicles, enabling them to perceive, interpret, and respond to the environment in real-time. The applications of deep learning in autonomous vehicles are vast and diverse, ranging from perception and object detection to decision-making and navigation. In this section, we will explore some of the key applications of deep learning in autonomous vehicles.
1 Perception and Object Detection
One of the primary applications of deep learning in autonomous vehicles is perception and object detection. Deep learning algorithms, such as convolutional neural networks (CNNs), are used to detect and classify objects in the vehicle's surroundings, including pedestrians, vehicles, traffic signs, and obstacles. By analyzing sensor data from cameras, LiDAR, and radar, deep learning models can accurately identify and track objects in real-time, enabling the vehicle to make informed decisions and navigate safely.
2 Localization and Mapping
Another important application of deep learning in autonomous vehicles is localization and mapping. Deep learning techniques, such as simultaneous localization and mapping (SLAM), are used to create high-definition maps of the vehicle's environment and accurately determine its position and orientation within that environment. By fusing data from sensors and GPS, deep learning models can improve the vehicle's localization accuracy and enable precise navigation in complex urban environments.
3 Path Planning and Decision-Making
Deep learning also plays a crucial role in path planning and decision-making for autonomous vehicles. Deep reinforcement learning (DRL) algorithms are used to train models that can anticipate future scenarios, evaluate potential actions, and make decisions in real-time. By simulating different driving scenarios and learning from experience, deep learning models can optimize the vehicle's trajectory, avoid collisions, and navigate efficiently through traffic.
4 Human-Machine Interaction
In addition to perception and decision-making, deep learning is also used in human-machine interaction for autonomous vehicles. Natural language processing (NLP) algorithms are deployed to enable seamless communication between the vehicle and passengers, allowing users to interact with the vehicle using voice commands or gestures. By understanding and responding to human input, deep learning models can enhance the overall user experience and improve the safety and comfort of autonomous driving.
5 Predictive Maintenance
Furthermore, deep learning is applied to predictive maintenance in autonomous vehicles. By analyzing sensor data and monitoring the vehicle's performance in real-time, deep learning models can anticipate potential mechanical failures or component malfunctions before they occur. By proactively identifying and addressing maintenance issues, deep learning can help optimize the vehicle's reliability, minimize downtime, and reduce operating costs for autonomous vehicle fleets.
In conclusion, the applications of deep learning in autonomous vehicles are vast and continue to evolve rapidly as technology advances. From perception and object detection to decision-making and predictive maintenance, deep learning enables autonomous vehicles to operate efficiently, safely, and intelligently in diverse and dynamic environments. As research and development in deep learning progress, the capabilities and applications of autonomous vehicles are only expected to expand, paving the way for a future of autonomous driving that is safer, more efficient, and more convenient for all.
Challenges and Limitations
As impressive as the advancements in deep learning have been for autonomous vehicles and AI, there are still several challenges and limitations that need to be addressed before we can fully realize their potential. In this section, we will discuss some of the most prominent obstacles facing the widespread adoption of deep learning in autonomous vehicles.
Data Availability and Quality
One of the biggest challenges facing deep learning in autonomous vehicles is the availability and quality of data. Deep learning algorithms require vast amounts of labeled data to train effectively, and this can be a significant hurdle in the autonomous vehicle industry. While there is a wealth of data available, ensuring its quality and relevance to the task at hand is crucial for the success of deep learning models.
Generalization and Robustness
Another challenge is the ability of deep learning algorithms to generalize and be robust in real-world scenarios. Autonomous vehicles operate in a dynamic and unpredictable environment, and deep learning models must be able to adapt to new situations and scenarios quickly. Ensuring that deep learning algorithms are robust and can handle edge cases is essential for the safety and reliability of autonomous vehicles.
Interpretability and Explainability
Interpretability and explainability are crucial aspects of deep learning in autonomous vehicles. Deep learning algorithms are often seen as black boxes, making it challenging to understand how they arrive at a particular decision. This lack of interpretability can be a significant hurdle when it comes to regulatory approval and trust in autonomous vehicles. Improving the interpretability and explainability of deep learning models is essential for their widespread adoption.
Safety and Ethics
Safety and ethics are also significant challenges facing deep learning in autonomous vehicles. Ensuring that autonomous vehicles operate safely and ethically is paramount, and deep learning algorithms must be designed with these considerations in mind. Addressing issues such as bias, fairness, and accountability is crucial for the responsible deployment of autonomous vehicles powered by deep learning.
Computational Resources
The computational resources required to train and deploy deep learning models in autonomous vehicles can be substantial. Deep learning algorithms are computationally intensive, and autonomous vehicles must have the necessary hardware and infrastructure to support them. Optimizing deep learning algorithms for efficiency and ensuring that autonomous vehicles have access to the required computational resources are critical challenges that need to be addressed.
Regulations and Standards
Regulations and standards surrounding deep learning in autonomous vehicles are still evolving. Ensuring compliance with existing regulations and shaping new standards that safeguard the safety and reliability of autonomous vehicles powered by deep learning is essential. Collaboration between industry stakeholders and regulatory bodies is crucial for establishing a framework that promotes innovation while prioritizing safety and ethical considerations.
In conclusion, while deep learning holds tremendous promise for autonomous vehicles and AI, addressing the challenges and limitations outlined in this section is essential for realizing their full potential. By tackling these obstacles head-on and working collaboratively towards innovative solutions, we can overcome the hurdles standing in the way of a future where autonomous vehicles powered by deep learning revolutionize transportation and redefine the way we interact with our surroundings.
Future Trends
As technology continues to advance at a rapid pace, the future of autonomous vehicles and AI is looking bright. There are several key trends that are expected to shape the future of deep learning in autonomous vehicles:
Enhanced Safety Features
One of the most important trends in the future of autonomous vehicles is the development of enhanced safety features. Deep learning algorithms are being used to improve the detection and recognition of objects on the road, helping to reduce the number of accidents and fatalities.
Improved Efficiency
Another trend in the future of autonomous vehicles is the focus on improving efficiency. Deep learning algorithms are being used to optimize routes, reduce energy consumption, and improve overall performance. This will help to make autonomous vehicles more cost-effective and sustainable in the long run.
Enhanced User Experience
As autonomous vehicles become more commonplace, there will be a growing demand for a more seamless and intuitive user experience. Deep learning algorithms can help to personalize the driving experience, adapt to the preferences of the user, and provide a more comfortable and enjoyable ride.
Integration with Smart Cities
The future of autonomous vehicles is closely tied to the development of smart cities. Deep learning algorithms can help autonomous vehicles communicate with infrastructure and other vehicles on the road, leading to a more connected and efficient transportation system.
Continued Research and Development
As deep learning technology continues to evolve, there will be ongoing research and development efforts to further improve the capabilities of autonomous vehicles. This will involve exploring new algorithms, collecting more data, and testing new technologies to push the boundaries of what is possible.
Overall, the future of deep learning in autonomous vehicles looks promising. With continued advancements in technology and a growing focus on safety, efficiency, and user experience, autonomous vehicles are on track to revolutionize the way we travel in the years to come.