The Power of Large Language Models in Artificial Intelligence
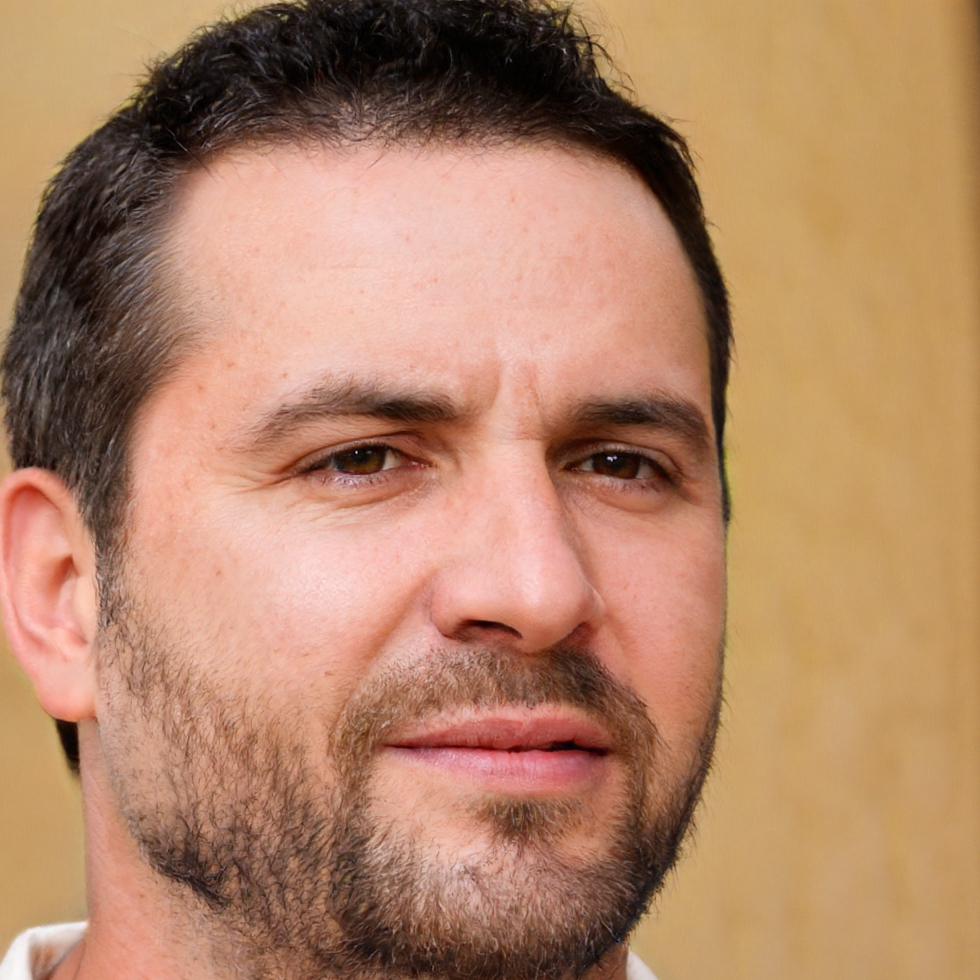
Author
Oliver ThompsonThis article explores the impact of large language models in the field of artificial intelligence. It delves into the significance of understanding these models, their various applications, as well as the challenges and limitations they present. Additionally, the article discusses the ethical implications of utilizing such models and offers insights into potential future directions in this rapidly evolving area of technology.
Introduction
Artificial Intelligence (AI) has seen significant advancements in recent years, with Large Language Models (LLMs) emerging as a prominent area of research and development. These models, powered by deep learning algorithms and vast amounts of data, have revolutionized the field of natural language processing (NLP) and are being applied across various industries and domains.
The rise of LLMs, such as OpenAI's GPT-3 and Google's BERT, has enabled machines to generate human-like text, engage in conversations, and perform a wide range of NLP tasks with remarkable accuracy. These models are trained on massive datasets, allowing them to learn the nuances of language and generate coherent and contextually relevant responses.
In this article, we will delve into the power of LLMs in AI and explore their capabilities, applications, challenges, limitations, and ethical implications. We will also discuss future directions in LLM research and development, highlighting the potential impact of these models on society and the way we interact with technology.
Understanding Large Language Models
Large Language Models, also known as LLMs, are a type of artificial intelligence technology that has gained significant attention in recent years. These models are designed to understand and generate human language using a vast amount of data and complex algorithms. They have the ability to process and produce text with remarkable accuracy, making them valuable tools for a wide range of applications.
At the core of LLMs is a neural network architecture that has been trained on a diverse range of text data, such as books, articles, and websites. This training process allows the model to learn the patterns and structures of language, enabling it to generate coherent and contextually relevant text. The size of LLMs can vary significantly, with some models containing billions of parameters that capture the nuances of human language.
One of the key components of LLMs is their ability to perform a variety of language-related tasks, such as text generation, translation, summarization, and sentiment analysis. These models can understand and generate text in multiple languages, making them versatile tools for communication and information processing. Additionally, LLMs have the capacity to learn from new data and adapt to changing contexts, allowing them to improve their performance over time.
The development of LLMs has been driven by advancements in deep learning and natural language processing techniques. Researchers and industry professionals have made significant progress in training larger and more powerful models that can handle complex language tasks with high accuracy. As a result, LLMs have become an integral part of many AI systems and applications, including chatbots, search engines, and content generation tools.
Despite their impressive capabilities, LLMs also face several challenges and limitations. For instance, these models may exhibit biases in their output due to the inherent biases present in the training data. Additionally, LLMs require substantial computational resources and data to train effectively, making them inaccessible to all researchers and organizations. Furthermore, LLMs may struggle with understanding and generating text in highly specialized or technical domains where data is limited.
In conclusion, LLMs represent a powerful and versatile technology that is transforming the field of artificial intelligence. By understanding the capabilities and limitations of these models, researchers and developers can harness their potential to create innovative solutions for a wide range of applications. As LLMs continue to evolve and improve, they will play a crucial role in shaping the future of AI and language processing.
Applications of Large Language Models
Large language models have revolutionized various fields and have opened up new possibilities for natural language processing tasks. These models can be applied in a wide range of applications, including but not limited to:
Text Generation
One of the most prominent applications of large language models is text generation. These models can generate coherent and contextually relevant text, making them ideal for tasks such as content creation, chatbots, automatic summarization, and storytelling.
Language Translation
Large language models have significantly improved language translation tasks by generating accurate and fluent translations between multiple languages. These models are used in machine translation services such as Google Translate and Microsoft Translator.
Sentiment Analysis
Large language models can also be used for sentiment analysis, where they analyze text data to determine the emotion or opinion expressed by the author. This application is essential for tasks such as social media monitoring, customer feedback analysis, and brand sentiment tracking.
Information Retrieval
Large language models can enhance information retrieval processes by understanding the context and intent behind user queries. These models can improve search engine results, question-answering systems, and knowledge graphs.
Personalization
Large language models enable personalization by generating tailored content and recommendations based on user preferences and behavior. These models power recommendation systems for e-commerce platforms, streaming services, and social media networks.
Dialogue Systems
Large language models are fundamental in building natural language understanding systems for dialogue. These systems can engage in conversations with users in a human-like manner, improving customer service chatbots, virtual assistants, and interactive voice response systems.
Content Moderation
Large language models can assist in content moderation by identifying and filtering out inappropriate or harmful content. These models are crucial for maintaining online safety and compliance with community guidelines on social media platforms and websites.
Semantic Search
Large language models support semantic search by understanding the meaning and context of search queries. This application improves search accuracy, relevance, and efficiency in information retrieval systems and search engines.
Overall, the applications of large language models are vast and continue to expand as researchers and developers explore new use cases in artificial intelligence and natural language processing.
Challenges and Limitations
Large language models, while powerful and versatile, are not without their challenges and limitations. In this section, we will discuss some of the key issues that researchers and developers face when working with these models.
1 Data and Bias
One of the biggest challenges with large language models is data and bias. These models are trained on vast amounts of text data, which can often contain biases and stereotypes. If not properly addressed, these biases can be perpetuated and amplified by the model, leading to unfair or discriminatory outputs. Ensuring that the training data is diverse and representative is crucial to mitigating this issue.
2 Computational Resources
Another challenge with large language models is the computational resources required to train and deploy them. Training a state-of-the-art model can be computationally intensive and expensive, requiring access to powerful hardware and large amounts of data. This can limit the accessibility of these models to researchers and organizations with limited resources.
3 Interpretability
Large language models are often referred to as "black boxes" due to their complexity and opacity. Understanding how these models arrive at their predictions can be difficult or impossible, making it challenging to interpret and trust their outputs. Improving the interpretability of these models is crucial for ensuring accountability and transparency.
4 Scalability
As language models continue to grow in size and complexity, scalability becomes a major concern. Scaling up these models can lead to diminishing returns, where the performance improvements plateau while the computational costs continue to increase. Finding the right balance between model size and performance is crucial for sustainable and efficient model development.
5 Fine-Tuning and Generalization
Fine-tuning large language models for specific tasks can be challenging due to generalization issues. While these models may perform well on benchmark datasets, they may struggle to generalize to unseen or out-of-domain data. Improving the generalization capabilities of these models is crucial for real-world applications.
6 Security and Privacy
Large language models raise security and privacy concerns due to their ability to generate politically sensitive or malicious content. Protecting against adversarial attacks and privacy breaches is essential to prevent misuse of these models and safeguard user data.
Overall, while large language models offer tremendous potential, addressing these challenges and limitations is essential for responsible and ethical deployment of these technologies.
Ethical Implications
The development and deployment of Large Language Models (LLMs) in artificial intelligence have raised several ethical implications that must be carefully considered. As these models become more powerful and sophisticated, the impact they have on society, individuals, and the environment grows significantly. It is crucial to assess and address these ethical implications in order to ensure that LLMs are used responsibly and ethically.
Privacy concerns
One of the primary ethical concerns surrounding LLMs is related to privacy. These models are trained on vast amounts of data, which often include personal information about individuals. There is a risk that this data could be misused or accessed without consent, leading to privacy violations. It is essential to establish strict guidelines and regulations to protect the privacy of individuals and prevent the misuse of personal data by LLMs.
Bias and discrimination
Another significant ethical issue with LLMs is the presence of bias and discrimination in the models. LLMs learn from the data they are trained on, which can often be biased or contain prejudices. This can result in the models producing biased outputs or reinforcing existing societal biases. It is critical to address and mitigate bias in LLMs to ensure fair and unbiased outcomes in their applications.
Misinformation and fake news
The spread of misinformation and fake news is a growing concern in the age of LLMs. These models have the ability to generate highly convincing and realistic text, making it difficult to distinguish between real and fake information. This can have serious implications for society, politics, and public discourse. It is important to implement mechanisms to detect and combat misinformation generated by LLMs.
Environmental impact
The training process for LLMs requires massive amounts of computational resources, which can have a significant environmental impact. The energy consumption and carbon footprint of training these models are substantial, contributing to climate change and environmental degradation. It is crucial to develop more sustainable and environmentally friendly training methods for LLMs to minimize their impact on the environment.
Accountability and transparency
Ensuring accountability and transparency in the development and deployment of LLMs is essential for maintaining trust and integrity in AI systems. It is important to establish clear guidelines and mechanisms for responsibility and accountability in the use of LLMs, as well as to provide transparency in their decision-making processes. This can help prevent misuse and unethical behavior in the deployment of LLMs.
In conclusion, the ethical implications of LLMs in artificial intelligence are diverse and complex. It is essential for researchers, developers, policymakers, and society as a whole to address these ethical concerns proactively and responsibly. By carefully considering and managing the ethical implications of LLMs, we can ensure that these powerful AI systems are used for the benefit of society while respecting the rights and values of individuals.
Future Directions
As large language models continue to advance in their capabilities and performance, there are several key areas where future research and development are likely to focus. These future directions encompass both technical advancements and broader societal considerations that will shape the evolution of artificial intelligence as a whole.
Improved Model Training
One key future direction for large language models is to enhance model training techniques to improve both efficiency and effectiveness. This may involve exploring new approaches to data augmentation, transfer learning, and regularization to better leverage the vast amounts of data available for training these models. Additionally, researchers may seek to develop novel optimization algorithms that can accelerate the training process without sacrificing model performance.
Enhanced Contextual Understanding
Another important future direction is to enhance models' ability to understand and utilize contextual information more effectively. This may involve developing better mechanisms for capturing long-range dependencies within text, as well as improving models' capabilities to reason and synthesize information across multiple sources. By enhancing contextual understanding, large language models could become even more adept at tasks such as natural language understanding, translation, and generation.
Addressing Bias and Fairness
A critical future direction for large language models is to address issues of bias and fairness in model development and deployment. Researchers are increasingly focusing on ways to mitigate bias in training data, as well as developing evaluation metrics to assess model fairness. This future direction may involve incorporating ethical considerations into the design and development of artificial intelligence systems, as well as promoting transparency and accountability in model deployment.
Scaling Up and Efficiency
As computing resources continue to advance, a key future direction for large language models is to further scale up models while maintaining efficiency. This may involve exploring novel architectures and algorithms that can handle even larger amounts of data and parameters, as well as optimizing model inference to reduce computational costs. By achieving greater scaling and efficiency, large language models could become even more versatile and applicable across a wide range of tasks and domains.
Bridging the Gap with Human Intelligence
One of the ultimate goals for large language models is to bridge the gap with human intelligence by achieving human-level performance on a variety of cognitive tasks. This future direction may involve developing hybrid models that combine the strengths of artificial intelligence with human expertise to tackle complex problems collaboratively. By bridging the gap with human intelligence, large language models could revolutionize fields such as medicine, education, and research, unlocking new capabilities and insights for society as a whole.
Collaboration and Interdisciplinary Research
In order to advance large language models to their full potential, collaboration and interdisciplinary research will be crucial future directions. This may involve partnerships between industry and academia, as well as cross-disciplinary collaborations with fields such as linguistics, cognitive science, and philosophy. By fostering collaboration and interdisciplinary research, large language models can draw upon a diverse range of perspectives and expertise, leading to innovative breakthroughs and transformative advancements in the field of artificial intelligence.
Overall, the future of large language models holds immense promise and potential for shaping the next generation of artificial intelligence. By focusing on these future directions, researchers and practitioners can pave the way for new and exciting developments that will push the boundaries of what AI can achieve in the years to come.