The Intersection of Machine Learning and Artificial Intelligence
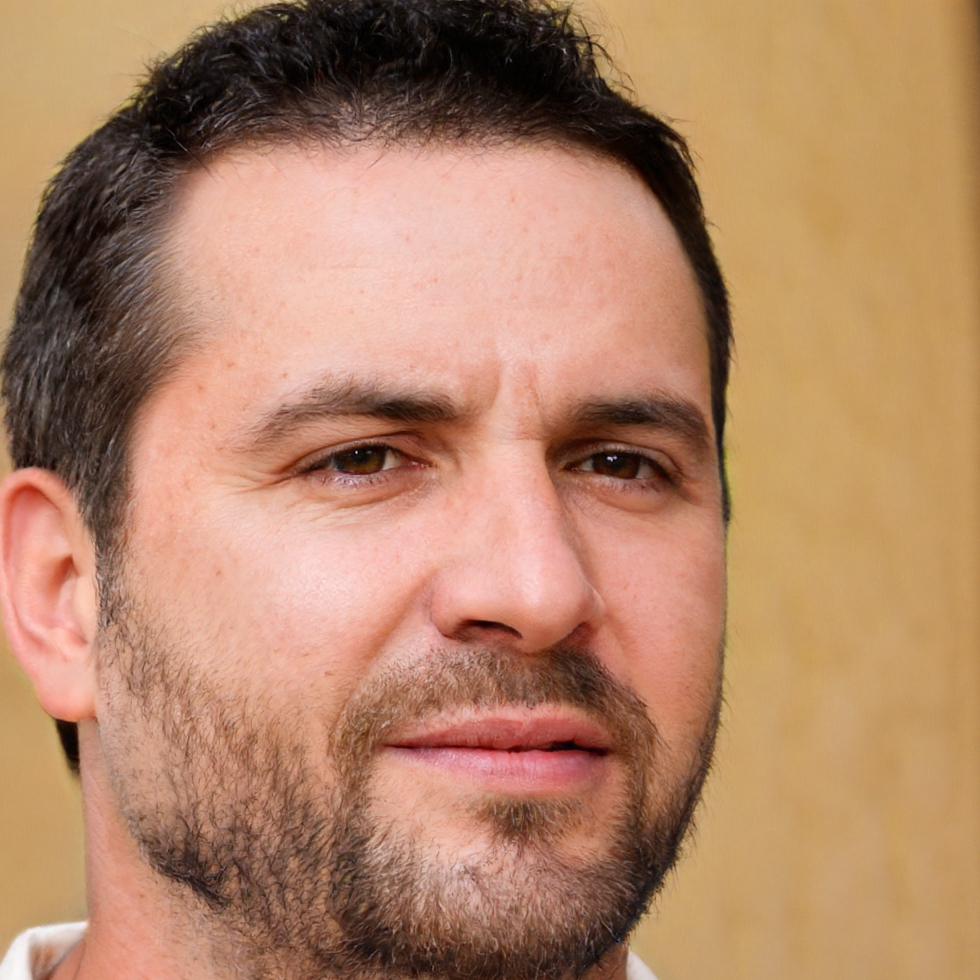
Author
Oliver ThompsonThe Intersection of Machine Learning and Artificial Intelligence explores the relationship between these two groundbreaking technologies. It delves into the definitions of Machine Learning and Artificial Intelligence, their applications in various industries such as Healthcare and Finance, as well as the Challenges and Limitations they face including Ethical Concerns and Bias. The article also looks towards the Future Trends in the field, including developments in Explainable AI and Automated Machine Learning.
Introduction
In the realm of technology and innovation, two terms that have gained significant attention in recent years are Machine Learning and Artificial Intelligence. These two fields have revolutionized various industries, from healthcare to finance, and have fundamentally changed the way we interact with digital systems.
Machine Learning, a subset of Artificial Intelligence, involves the development of algorithms and models that enable computers to learn from data. This means that instead of being explicitly programmed to perform a task, machines can use data to improve their performance over time. This iterative process allows machines to make predictions, detect patterns, and ultimately make decisions without human intervention.
Artificial Intelligence, on the other hand, refers to the broader concept of creating machines or systems that can perform tasks that typically require human intelligence. This encompasses a wide range of applications, from simple recommendation systems to complex autonomous vehicles. AI systems are designed to mimic human cognitive functions such as learning, reasoning, and problem-solving.
The intersection of Machine Learning and Artificial Intelligence represents the cutting edge of technological advancement. By leveraging data and algorithms, organizations can unlock new insights, optimize processes, and create innovative products and services. However, with great power comes great responsibility, as the adoption of ML and AI also raises ethical concerns and challenges related to bias and fairness.
In this article, we will delve into the definitions of Machine Learning and Artificial Intelligence, explore their applications in healthcare and finance, discuss the challenges and limitations they present, and look ahead to future trends in the field. Let's embark on a journey through the fascinating world of ML and AI and uncover their potential to shape the future of technology.
Understanding Machine Learning and Artificial Intelligence
In order to fully grasp the concept of Machine Learning (ML) and Artificial Intelligence (AI), it is essential to understand the differences and similarities between the two fields. Both ML and AI are revolutionizing various industries and driving innovation across the globe.
Definition of Machine Learning
Machine Learning can be defined as a subset of AI that focuses on enabling machines to learn from data without being explicitly programmed. In other words, ML algorithms use data to learn patterns and make predictions or decisions. ML models improve their performance over time as they are exposed to more data. There are three main types of ML: supervised learning, unsupervised learning, and reinforcement learning.
- Supervised Learning: In supervised learning, the algorithm is trained on labeled data, where the correct output is provided. The goal is to learn a mapping function from inputs to outputs.
- Unsupervised Learning: Unsupervised learning involves training the algorithm on unlabeled data. The algorithm learns patterns and relationships in the data without explicit guidance.
- Reinforcement Learning: Reinforcement learning is a type of ML where an agent learns to make decisions by interacting with an environment. The agent receives rewards or penalties based on its actions.
Definition of Artificial Intelligence
Artificial Intelligence refers to the simulation of human intelligence processes by machines, including learning, reasoning, problem-solving, perception, and language understanding. AI systems aim to mimic human cognitive functions and make autonomous decisions. AI can be categorized into two main types: narrow AI and general AI.
- Narrow AI: Narrow AI, also known as weak AI, is designed to perform specific tasks or solve particular problems. Examples of narrow AI include virtual assistants like Siri and Alexa, recommendation systems, and image recognition software.
- General AI: General AI, also called strong AI, refers to a machine's ability to understand and learn any intellectual task that a human can. General AI is still a theoretical concept and has not been achieved yet.
Both ML and AI work hand in hand to enable machines to perform tasks that require human intelligence. By leveraging data and advanced algorithms, ML and AI have the potential to transform industries and society as a whole.
Applications of Machine Learning and Artificial Intelligence
Machine Learning and Artificial Intelligence have a wide range of applications across various industries. They have the potential to revolutionize the way we live, work, and interact with technology. In this section, we will explore some of the key areas where these technologies are being utilized.
In Healthcare
In the field of healthcare, Machine Learning and Artificial Intelligence are being used to improve patient care, optimize hospital operations, and enhance medical research.
-
Diagnosis and Treatment: Machine Learning algorithms can analyze medical images, such as X-rays and MRIs, to assist doctors in diagnosing diseases and conditions more accurately and quickly. They can also help in predicting the effectiveness of treatments.
-
Personalized Medicine: By analyzing genetic and clinical data, Artificial Intelligence can help in the development of personalized treatment plans for patients. This tailored approach can lead to better outcomes and reduced side effects.
-
Drug Discovery: Machine Learning is being used to identify potential drug candidates and predict their efficacy. This has the potential to speed up the drug discovery process and bring new treatments to market faster.
In Finance
The financial industry is another sector where Machine Learning and Artificial Intelligence are making a significant impact. These technologies are being used to streamline operations, improve decision-making, and enhance customer experiences.
-
Fraud Detection: Machine Learning algorithms can analyze large volumes of financial data to detect patterns and anomalies that may indicate fraudulent activities. This can help financial institutions to prevent fraud and protect their customers.
-
Risk Management: By analyzing market data and economic trends, Artificial Intelligence can help in predicting and mitigating risks in financial investments. This can lead to more informed decision-making and better portfolio management.
-
Customer Service: Chatbots powered by Machine Learning are being used by financial institutions to provide personalized customer service and support. These chatbots can answer customer queries, provide account information, and even assist in financial planning.
In conclusion, the applications of Machine Learning and Artificial Intelligence in healthcare and finance are just a few examples of how these technologies are transforming industries and improving outcomes for individuals and businesses. The potential for innovation and growth in this field is immense, and we can expect to see even more exciting developments in the future.
Challenges and Limitations
One of the key challenges facing the intersection of Machine Learning (ML) and Artificial Intelligence (AI) is the issue of Ethical Concerns. With the increasing use of AI and ML technologies in various industries, there is a growing need to address the ethical implications of these technologies.
One major concern is the potential for AI algorithms to reinforce existing biases. In many cases, AI systems are trained on data that may contain biases, which can result in discriminatory outcomes. For example, biased data used to train a hiring algorithm could lead to the exclusion of certain groups from job opportunities. Addressing bias in AI systems is a complex challenge that requires careful attention to data collection, preprocessing, and model training.
Another ethical concern is the lack of transparency in AI systems. Many AI models operate as "black boxes," meaning that their decision-making processes are not easily understandable or explainable. This lack of transparency can lead to issues of accountability and trust, as users may not be able to understand how AI systems are making decisions.
In addition to ethical concerns, there are also technical limitations facing the field of ML and AI. One significant limitation is the issue of scalability. Many ML algorithms require large amounts of computational resources and data to train effectively. As a result, deploying ML models at scale can be challenging, particularly for resource-constrained organizations.
Another technical limitation is the need for continuous learning. ML models are typically trained on historical data, which means they may not always be able to adapt to new or evolving trends. This can be a significant challenge in dynamic environments where data is constantly changing.
Overall, addressing the ethical concerns and technical limitations of ML and AI will be critical in ensuring the responsible and effective use of these technologies in the future. By developing more transparent and accountable AI systems and overcoming scalability and adaptability challenges, we can harness the potential of ML and AI to drive innovation and positive change in society.
Future Trends
Artificial Intelligence (AI) and Machine Learning (ML) are rapidly evolving fields with numerous potential applications and implications for society. As technology continues to advance, it is important to keep an eye on the future trends that will shape the development of AI and ML. In this section, we will discuss some of the key trends that are likely to emerge in the near future.
Explainable AI
One of the main challenges in the adoption of AI systems is the lack of transparency in how these systems make decisions. As AI becomes more widespread in various industries, there is a growing demand for explainable AI solutions. Explainable AI refers to the ability of AI systems to provide understandable explanations for their decisions and actions. This is crucial for ensuring accountability and trust in AI systems, especially in fields where ethical concerns are paramount, such as healthcare and finance.
The development of explainable AI techniques will not only help improve the interpretability of AI systems but also enable users to better understand and validate the results produced by these systems. This will lead to greater reliability and acceptance of AI technologies across different sectors.
Automated Machine Learning
Another key trend in the field of AI and ML is automated machine learning (AutoML). AutoML refers to the use of automated tools and algorithms to streamline the process of building and deploying machine learning models. With the increasing complexity and volume of data available today, there is a growing need for efficient and scalable solutions to model development.
AutoML solutions aim to automate various aspects of the machine learning workflow, including data preprocessing, feature selection, hyperparameter tuning, and model evaluation. By automating these tasks, AutoML can help reduce the time and resources required to develop high-quality ML models, making it easier for non-experts to leverage the power of AI in their applications.
In conclusion, the future trends in AI and ML are poised to transform the way we interact with technology and drive innovation across various industries. By embracing these trends and staying up-to-date with the latest developments in the field, we can harness the full potential of AI and ML to create a better future for all.