The Future Impact of NLP on Artificial Intelligence
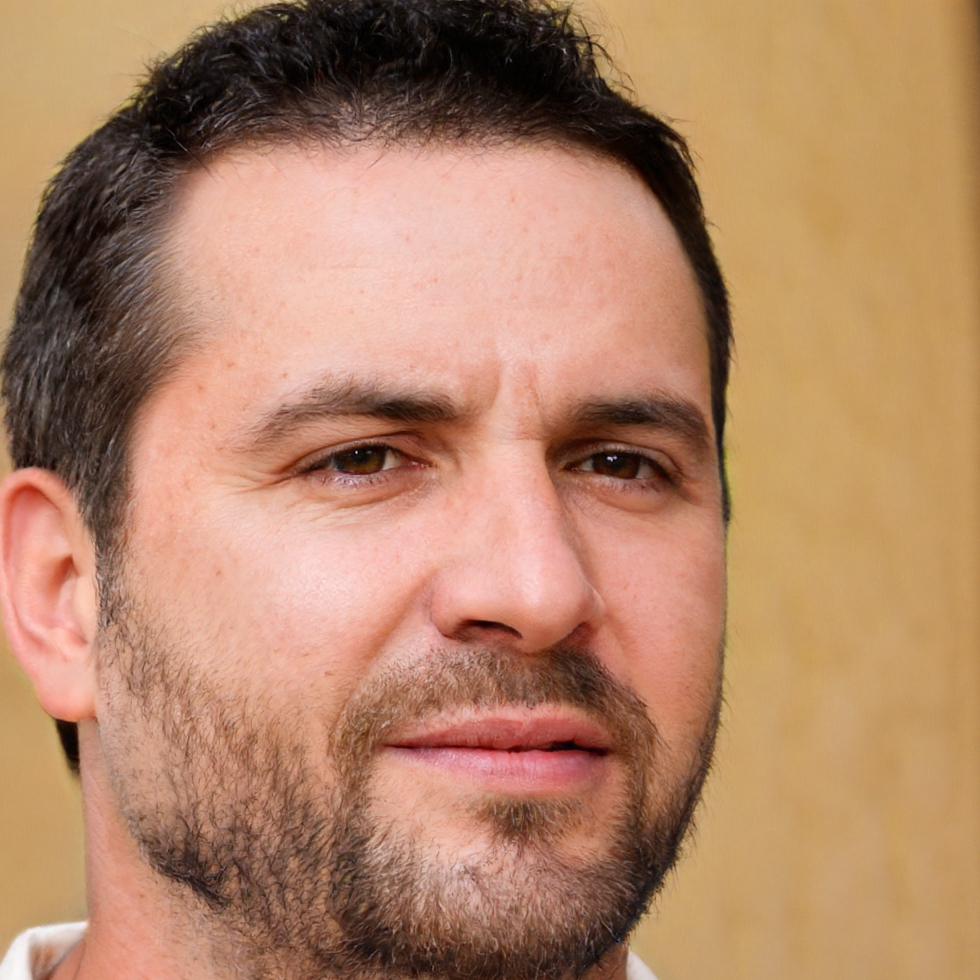
Author
Oliver ThompsonNatural Language Processing (NLP) has emerged as a crucial component in the field of Artificial Intelligence (AI), enabling machines to understand and process human language. This article delves into the history of NLP, the current applications of NLP in AI, the challenges faced in NLP for AI, advancements in NLP technology, future possibilities for NLP in AI, and the ethical implications of incorporating NLP into AI systems. Follow along to explore how NLP is shaping the future of AI.
Introduction
Natural Language Processing (NLP) has emerged as a crucial component in the field of Artificial Intelligence (AI) in recent years, revolutionizing the way machines interact with human language. NLP refers to the ability of a computer program to understand, interpret, and generate human language in a way that is both meaningful and useful. This technology has vast implications for a wide range of industries, from customer service and healthcare to education and marketing.
The use of NLP in AI has grown exponentially with the rise of chatbots, virtual assistants, sentiment analysis, language translation, and more. These applications have made it possible for machines to communicate with humans in a more natural and intuitive way. As NLP continues to advance, its impact on AI is expected to further enhance the capabilities of machines to comprehend and respond to human language.
This article will explore the history of NLP and its evolution within the field of AI. It will also examine the current applications of NLP in AI and the challenges that researchers and developers face in improving these technologies. Furthermore, this article will highlight the recent advancements in NLP technology and the potential future possibilities for NLP in AI. Additionally, we will discuss the ethical implications of using NLP in AI and how society may need to address these concerns moving forward.
Overall, this article aims to provide a comprehensive overview of the role of NLP in AI and its potential impact on the future of technology and human-machine interaction. We will delve into the intricacies of NLP and the exciting possibilities that lie ahead as we continue to push the boundaries of artificial intelligence.
History of Natural Language Processing (NLP)
Natural Language Processing (NLP) has a rich history that spans several decades. The field of NLP can be traced back to the 1950s when researchers first began exploring ways to enable computers to understand and generate human language. Over the years, NLP has evolved significantly, driven by advancements in artificial intelligence and computational linguistics.
Early Developments in NLP
In the early days of NLP, researchers focused on basic tasks such as language translation, information retrieval, and speech recognition. These early systems were limited in their capabilities and often struggled to accurately interpret the nuances of human language.
One of the key milestones in the history of NLP was the development of the Shannon-Weaver model in the 1940s, which laid the foundation for computational linguistics. This model introduced the concept of information theory and paved the way for future advancements in NLP.
Breakthroughs in the 20th Century
The field of NLP saw significant progress in the latter half of the 20th century, with researchers making breakthroughs in areas such as syntax, semantics, and pragmatics. In 1954, the Georgetown-IBM experiment demonstrated the potential for machine translation, marking a major milestone in the history of NLP.
Throughout the 1960s and 1970s, researchers continued to refine NLP systems, developing techniques such as parsing algorithms and semantic analysis. These advancements laid the groundwork for more sophisticated language processing capabilities in the years to come.
Rise of Machine Learning in NLP
In recent decades, the field of NLP has been revolutionized by the rise of machine learning and deep learning. Researchers have leveraged these techniques to develop neural networks and statistical models that can analyze and generate human language with unprecedented accuracy.
The emergence of big data and cloud computing has further accelerated progress in NLP, enabling researchers to train more robust language models and translation systems. Today, NLP is used in a wide range of applications, from virtual assistants and chatbots to sentiment analysis and automated content generation.
Overall, the history of NLP is marked by a series of key developments and breakthroughs that have shaped the field into what it is today. As technology continues to advance, the future of NLP in artificial intelligence holds great promise for further innovation and growth.
Current Applications of NLP in AI
Natural Language Processing (NLP) has made significant advancements in recent years, leading to a wide range of applications in Artificial Intelligence (AI). NLP technology allows computers to understand, interpret, and generate human language, enabling them to interact with humans in a more natural way. The following are some of the current applications of NLP in AI:
Sentiment Analysis
Sentiment analysis is a popular application of NLP that involves analyzing and understanding people's opinions, attitudes, and emotions expressed in text data. Businesses use sentiment analysis to gauge customer satisfaction, monitor brand reputation, and understand trends in social media conversations. NLP techniques are employed to automatically classify text as positive, negative, or neutral based on the sentiment expressed.
Chatbots and Virtual Assistants
Chatbots and virtual assistants are AI applications that use NLP to interact with users through natural language conversations. These tools can help users find information, answer questions, schedule appointments, and perform various tasks through text or voice commands. NLP algorithms enable chatbots to understand user queries, extract relevant information, and respond in a human-like manner.
Machine Translation
Machine translation is another key application of NLP that involves automatically translating text from one language to another. Online platforms like Google Translate use NLP algorithms to understand the context and nuances of different languages, allowing for accurate and fluent translations. NLP techniques help improve translation quality by considering grammar rules, idiomatic expressions, and cultural nuances.
Text Summarization
Text summarization is a useful NLP application that involves condensing large amounts of text into shorter versions while retaining the most important information. This technology is valuable for generating summaries of news articles, research papers, and legal documents. NLP models can extract key sentences, identify essential information, and create concise summaries that capture the main points of the original text.
Named Entity Recognition
Named Entity Recognition (NER) is an NLP task that involves identifying and classifying named entities in text data, such as names of people, organizations, locations, dates, and more. NER algorithms are used in various applications, including information retrieval, document classification, and entity linking. NLP techniques enable machines to accurately recognize and extract named entities from unstructured text.
Speech Recognition
Speech recognition technology uses NLP algorithms to convert spoken language into written text, allowing for hands-free communication and voice-controlled devices. Virtual assistants like Amazon Alexa, Apple Siri, and Google Assistant rely on speech recognition to understand user commands, transcribe audio recordings, and perform actions based on voice input. NLP advancements have improved the accuracy and efficiency of speech recognition systems.
Text Classification
Text classification is a fundamental NLP task that involves categorizing text data into predefined classes or categories. This application is used in email filtering, sentiment analysis, document categorization, and spam detection. NLP models can analyze text content, extract features, and classify documents based on their content and context. Text classification algorithms are essential for organizing and managing large volumes of textual data.
Question Answering Systems
Question answering systems utilize NLP techniques to automatically generate answers to user questions based on a given context or knowledge base. These systems can extract relevant information from textual sources, understand the query semantics, and provide accurate responses in natural language. Question answering applications are used in customer support, educational platforms, and information retrieval systems to assist users in finding specific information.
Content Recommendation
Content recommendation systems leverage NLP technology to analyze user preferences, behavior, and interaction patterns to suggest personalized content. Platforms like Netflix, Spotify, and Amazon use NLP algorithms to recommend movies, music, products, and articles based on user interests and past interactions. NLP models can understand user feedback, interpret content relevance, and enhance user engagement by delivering tailored recommendations.
In conclusion, the current applications of NLP in AI are diverse and impactful, empowering machines to understand and generate human language with increasing accuracy and efficiency. As NLP technology continues to advance, we can expect to see further innovation and integration of NLP in various AI applications, shaping the future of human-computer interaction and communication.
Challenges in NLP for AI
Natural Language Processing (NLP) has made significant advancements in recent years, but there are still challenges that researchers and developers need to address in order to further improve its capabilities within the field of Artificial Intelligence (AI). Some of the key challenges in NLP for AI include:
Data Quality and Quantity
One of the biggest challenges in NLP is the availability of high-quality training data. NLP models require large amounts of data to learn the nuances of human language, but sourcing and labeling this data can be time-consuming and costly. Additionally, bias in training data can perpetuate stereotypes and lead to inaccurate or unfair results.
Ambiguity and Context
Human language is inherently ambiguous and context-dependent. NLP models often struggle with understanding the correct meaning of a word or phrase based on the surrounding context. Resolving ambiguity and improving contextual understanding are ongoing challenges in NLP research.
Syntax and Grammar
Parsing and analyzing the syntax and grammar of natural language texts is a complex task for NLP models. Languages can have variations in grammar rules, sentence structures, and word order, making it difficult for AI systems to accurately interpret and generate language.
Multilingualism
Another challenge in NLP is developing multilingual models that can effectively process and generate text in multiple languages. Translating between languages, understanding language dialects, and handling code-switching are all areas that require further research and development.
Real-time Processing
NLP applications often need to process and generate text in real-time, such as in chatbots, virtual assistants, and automated translation services. Achieving low latency and high accuracy in real-time NLP tasks remains a challenge due to the computational complexity of language processing.
Explainability and Interpretability
As NLP models become more powerful and complex, there is a growing need for explainable and interpretable AI systems. Understanding how NLP models arrive at their predictions and decisions is crucial for ensuring transparency and accountability in AI applications.
Privacy and Security
Protecting user privacy and securing sensitive data are critical considerations in NLP applications, especially in tasks involving personal information or confidential data. Developing robust privacy-preserving techniques and security measures is essential in the continued advancement of NLP in AI.
Adaptability and Generalization
NLP models often struggle to generalize to new tasks or adapt to unseen data. Achieving robustness and flexibility in NLP models is a key challenge for improving their performance across a wide range of language tasks and domains.
In conclusion, addressing these challenges in NLP for AI will require collaborative efforts from researchers, developers, policymakers, and industry stakeholders. Overcoming these challenges will not only advance the capabilities of NLP technology but also pave the way for more ethical, inclusive, and sustainable applications of AI in natural language processing.
Advancements in NLP Technology
Natural Language Processing (NLP) has seen significant advancements in recent years, thanks to the rapid growth of machine learning and artificial intelligence technologies. These advancements have enabled NLP to become more accurate, efficient, and versatile in handling a wide range of natural language tasks. In this section, we will discuss some of the key advancements in NLP technology that are shaping the future of artificial intelligence.
Neural Networks and Deep Learning
One of the most significant advancements in NLP technology is the widespread adoption of neural networks and deep learning techniques. These methods have revolutionized how NLP systems process and analyze large amounts of text data, leading to improvements in tasks such as machine translation, sentiment analysis, and speech recognition. Deep learning models, such as Recurrent Neural Networks (RNNs) and Transformer architectures, have shown impressive results in capturing complex patterns in natural language data.
Transfer Learning and Pre-trained Models
Another major advancement in NLP technology is the use of transfer learning and pre-trained models. By leveraging large-scale language models like BERT (Bidirectional Encoder Representations from Transformers) and GPT (Generative Pre-trained Transformer), NLP systems can learn from vast amounts of text data and transfer this knowledge to new tasks with minimal fine-tuning. This has significantly improved the performance of NLP models on various natural language processing tasks.
Multimodal NLP
Multimodal NLP, which integrates information from different modalities such as text, images, and audio, has emerged as a key area of advancement in NLP technology. By combining multiple sources of information, multimodal NLP systems can perform more sophisticated natural language understanding tasks, such as image captioning, visual question answering, and audio transcription. This fusion of modalities has opened up new possibilities for NLP applications in various domains.
Contextual Understanding and Reasoning
Advancements in NLP technology have also focused on enhancing contextual understanding and reasoning capabilities in natural language systems. Models like BERT and GPT have shown remarkable progress in capturing contextual information and generating coherent responses in conversational settings. These advancements are crucial for improving the overall intelligence and naturalness of NLP systems.
Interdisciplinary Research and Collaboration
The field of NLP has seen increased interdisciplinary research and collaboration with other domains such as cognitive science, linguistics, and psychology. By incorporating insights from these disciplines, NLP researchers are gaining a deeper understanding of how natural language is processed and interpreted by humans, leading to more robust and effective NLP systems. This interdisciplinary approach is driving further advancements in NLP technology.
In conclusion, the recent advancements in NLP technology have significantly expanded the capabilities of artificial intelligence systems in processing and understanding natural language. From neural networks and deep learning to transfer learning and multimodal NLP, these advancements are paving the way for more sophisticated and intelligent NLP applications in various industries and domains. As research in NLP continues to evolve, we can expect even more groundbreaking developments that will shape the future of artificial intelligence.
Future Possibilities for NLP in AI
The field of Natural Language Processing (NLP) has seen significant advancements in recent years, leading to exciting possibilities for its integration with Artificial Intelligence (AI). As technology continues to evolve, the future of NLP in AI holds great promise for various industries and applications. Some of the key future possibilities for NLP in AI include:
Voice Assistants and Virtual Agents
One of the most prominent applications of NLP in AI is the development of voice assistants and virtual agents. These intelligent systems can understand and respond to human language, enabling seamless interactions between users and machines. The future of NLP in AI will likely see the continued improvement of voice assistants, making them more accurate, context-aware, and personalized.
Language Translation and Multilingual Communication
NLP has already revolutionized the way we communicate across languages, with real-time translation technologies becoming increasingly sophisticated. In the future, NLP in AI could enable instantaneous and natural communication between individuals speaking different languages, breaking down language barriers and fostering global collaboration.
Sentiment Analysis and Emotional Intelligence
With advancements in sentiment analysis and emotion recognition, NLP in AI is poised to develop emotional intelligence. Future systems may be able to detect and respond to human emotions, enhancing customer experiences, personalized interactions, and mental health support.
Content Generation and Personalized Recommendations
NLP in AI can empower content creators and marketers by automating the process of content generation and delivering personalized recommendations to users. In the future, we may see AI-powered writers, social media algorithms, and recommendation engines becoming more sophisticated and effective through NLP.
Healthcare and Medical Diagnosis
In the healthcare industry, NLP in AI holds the potential to revolutionize medical diagnosis and patient care. By analyzing medical records, symptoms, and research data, AI-powered systems could assist healthcare professionals in making accurate diagnoses, prescribing tailored treatments, and improving patient outcomes.
Enhanced Conversational Interfaces and Human-Machine Interaction
The future of NLP in AI will likely see the development of more human-like and intuitive conversational interfaces, enabling natural conversations between humans and machines. These advanced interfaces could be used in customer service, virtual reality, education, and entertainment, providing engaging and immersive experiences.
Overall, the future possibilities for NLP in AI are vast and exciting, with the potential to transform the way we interact with technology, communicate with each other, and solve complex problems. By harnessing the power of NLP in AI, we can unlock unprecedented capabilities and innovations that will shape the future of artificial intelligence.
Ethical Implications of NLP in AI
As Natural Language Processing (NLP) continues to advance and integrate with Artificial Intelligence (AI), there are a number of ethical considerations that must be taken into account. These ethical implications span a wide range of areas, including privacy, bias, authenticity, accountability, and transparency. It is crucial for developers, policymakers, and the broader society to actively engage in discussions about the ethical ramifications of NLP in AI to ensure that these technologies are used responsibly and ethically.
Privacy Concerns
One of the primary ethical concerns surrounding NLP in AI is the issue of privacy. As NLP systems become more adept at processing and understanding human language, there is a risk that sensitive information could be inadvertently disclosed or misused. For example, chatbots and virtual assistants that utilize NLP may store and analyze conversations, raising questions about data security and user consent. It is essential for organizations to implement robust privacy practices and ensure that user data is protected from misuse.
Bias and Discrimination
Another significant ethical challenge is the presence of bias in NLP systems. These biases can arise from the data used to train the models, as well as the algorithms themselves. If not addressed, bias in NLP can perpetuate discrimination against certain groups or reinforce existing societal inequalities. It is important for developers to actively mitigate bias in NLP systems through careful data selection, algorithmic transparency, and ongoing monitoring.
Authenticity and Misinformation
NLP technologies have the potential to manipulate and generate text in ways that could deceive or mislead individuals. This raises concerns about the authenticity of content produced by NLP systems, as well as the spread of misinformation. As AI-generated text becomes more indistinguishable from human-written text, it becomes increasingly important to develop mechanisms for verifying the authenticity of information and combatting the spread of false or misleading content.
Accountability and Transparency
The deployment of NLP in AI raises questions about accountability and transparency. When AI systems make decisions based on NLP analysis, it may be challenging to determine who is responsible for the outcomes. Additionally, the inner workings of NLP algorithms can be complex and opaque, making it difficult to understand how decisions are made. It is essential for organizations to establish clear lines of accountability and provide transparency into the functioning of NLP systems to ensure that decisions are fair and unbiased.
Regulation and Governance
Given the potential ethical challenges associated with NLP in AI, there is a growing need for regulation and governance. Policymakers and regulators must work to develop frameworks that promote ethical NLP practices and protect individuals from potential harms. This may include establishing guidelines for data collection and use, implementing safeguards against bias and discrimination, and creating mechanisms for accountability and oversight.
In conclusion, the ethical implications of NLP in AI are complex and multifaceted. To harness the full potential of these technologies while minimizing risks, it is essential for stakeholders to engage in ongoing dialogue and collaboration to address these ethical challenges. By prioritizing ethics and responsible use, we can ensure that NLP technologies contribute positively to society and uphold fundamental values such as privacy, fairness, and transparency.