Exploring the Power of NLP in Artificial Intelligence
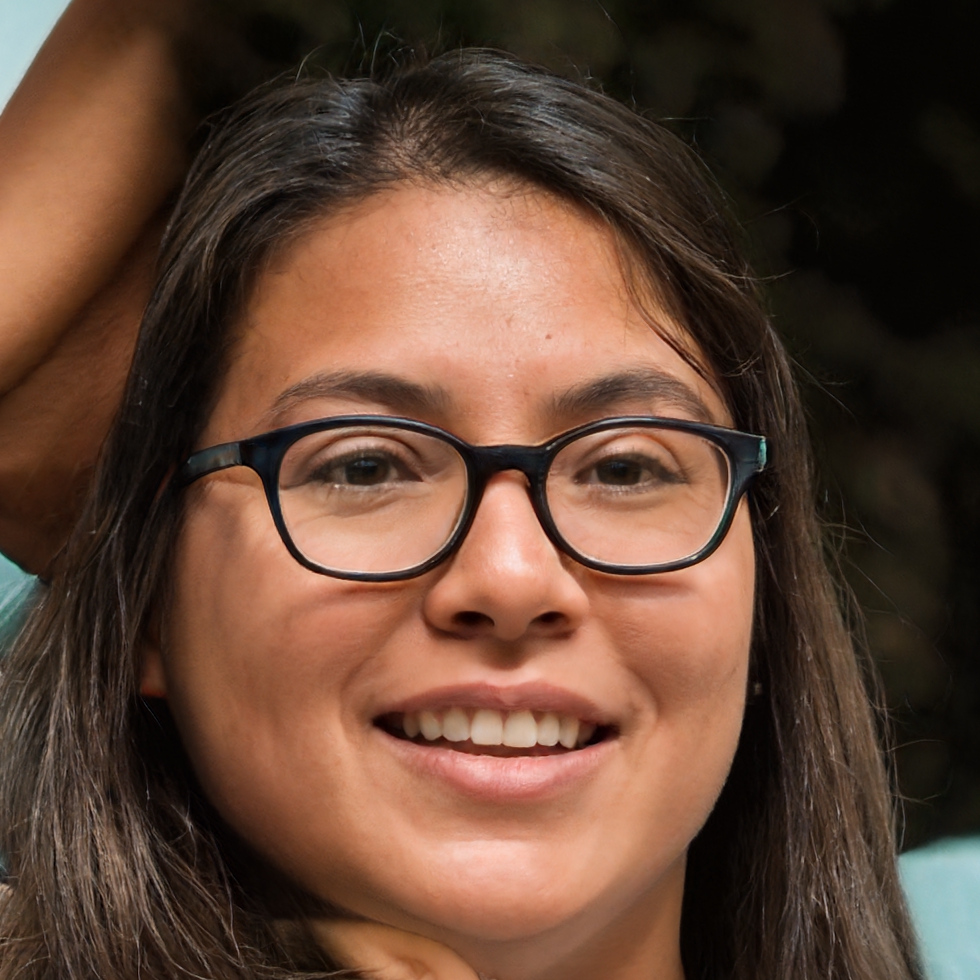
Author
Isabella HernandezThis article delves into the power of Natural Language Processing (NLP) in the realm of Artificial Intelligence (AI). It explores the definition of NLP in AI, its various applications, the challenges faced in its implementation, and the future trends that are shaping the intersection of NLP and AI technologies.
Introduction
Natural Language Processing (NLP) has emerged as a crucial component in the field of Artificial Intelligence (AI), revolutionizing the way machines interact with humans. NLP technology enables computers to understand, interpret, and generate human language, allowing for seamless communication between man and machine.
With the rapid advancements in AI, NLP has become a powerful tool that drives various applications such as speech recognition, language translation, sentiment analysis, chatbots, and more. This has opened up a world of possibilities in industries ranging from healthcare and finance to marketing and customer service.
In this comprehensive article, we will explore the power of NLP in Artificial Intelligence, examining its definition, applications, challenges, and future trends. We will delve deep into the intricacies of NLP technology and its impact on shaping the future of AI. Join us on this journey as we unravel the fascinating world of NLP and its role in driving innovation and transformation in the ever-evolving landscape of AI.
What is NLP in Artificial Intelligence?
Natural Language Processing (NLP) is a branch of artificial intelligence (AI) that focuses on the interaction between computers and humans using natural language. The goal of NLP is to enable computers to understand, interpret, and generate human language in a way that is both natural and meaningful.
At its core, NLP involves a combination of linguistics, computer science, and artificial intelligence. By leveraging these disciplines, NLP algorithms can analyze large amounts of natural language data, extract key insights, and generate responses that mimic human language.
One of the key components of NLP is natural language understanding (NLU), which involves the comprehension of natural language input. NLU algorithms are designed to process and analyze text or speech inputs, identify key elements such as entities and context, and derive meaning from the input.
Another important aspect of NLP is natural language generation (NLG), which focuses on generating human-like language output. NLG algorithms can generate coherent and contextually relevant responses to text or speech inputs, allowing computers to communicate with humans in a way that feels natural and authentic.
In the context of AI, NLP plays a crucial role in enabling machines to interact with humans in a more intuitive and efficient manner. From chatbots and virtual assistants to automated translation services and sentiment analysis, NLP is powering a wide range of AI applications that rely on natural language input and output.
Overall, NLP in artificial intelligence is a rapidly advancing field that continues to push the boundaries of what machines can achieve in terms of understanding and generating natural language. As NLP technology continues to evolve, we can expect to see even more sophisticated and context-aware AI systems that are capable of engaging with humans in more personalized and meaningful ways.
Applications of NLP in AI
Natural Language Processing (NLP) has a wide range of applications in Artificial Intelligence (AI) that have revolutionized various industries. Some of the key applications of NLP in AI include:
1. Sentiment Analysis
One of the most common applications of NLP in AI is sentiment analysis. By analyzing text data from social media, customer reviews, and other sources, NLP algorithms can determine the sentiment or emotion behind the text. This can be valuable for businesses looking to understand how customers feel about their products or services, as well as for monitoring online reputation and brand sentiment.
2. Chatbots and Virtual Assistants
NLP is also widely used in the development of chatbots and virtual assistants. These AI-powered tools are designed to interact with users in natural language, answering questions, providing information, and completing tasks. By leveraging NLP, chatbots can understand and interpret user inputs, carry on conversations, and provide personalized responses.
3. Language Translation
NLP technologies have significantly improved language translation capabilities, enabling users to translate text from one language to another with a high level of accuracy. Machine translation tools such as Google Translate and Microsoft Translator utilize NLP algorithms to analyze and translate text, making it easier for people around the world to communicate and access information in multiple languages.
4. Text Classification
NLP is used in text classification tasks, such as categorizing documents, emails, and social media posts into predefined categories or topics. By analyzing the content of text data, NLP algorithms can automatically classify and organize information, making it easier to search, retrieve, and analyze large volumes of text.
5. Information Extraction
Another important application of NLP in AI is information extraction. NLP algorithms can extract specific information and relationships from unstructured text data, such as named entities, keywords, and semantic relationships. This can be useful for various tasks, including data mining, knowledge discovery, and content analysis.
6. Speech Recognition
NLP is also utilized in speech recognition systems that can transcribe spoken language into text. By converting audio input into text data, NLP algorithms enable users to interact with devices and applications using voice commands. Speech recognition technology is used in virtual assistants, dictation software, and other speech-enabled applications.
7. Content Generation
NLP algorithms can be applied to generate human-like text content, such as articles, stories, and product descriptions. By analyzing existing text data, NLP models can learn to mimic the style, tone, and structure of the original content, producing new text that is coherent and meaningful. Content generation with NLP is used in various applications, including content marketing, automated writing, and personalized recommendations.
Overall, the applications of NLP in AI are diverse and impactful, offering numerous opportunities for innovation and advancement in various domains. With the continued development of NLP technologies and AI capabilities, the potential for leveraging natural language processing in real-world applications continues to grow.
Challenges in Implementing NLP in AI
Implementing Natural Language Processing (NLP) in Artificial Intelligence (AI) comes with its own set of challenges. While NLP has made significant advancements in recent years, there are still several obstacles that need to be overcome in order to fully harness its power in AI applications. Some of the major challenges in implementing NLP in AI include:
Data Quality and Quantity
One of the biggest challenges in implementing NLP in AI is the availability of high-quality data. NLP algorithms require large amounts of text data to train on, and ensuring that this data is accurate and diverse is critical for the success of NLP models. Additionally, obtaining labeled data for training can be a time-consuming and expensive process, which can hinder the development of NLP applications.
Ambiguity and Context
Natural language is inherently ambiguous and context-dependent, making it difficult for NLP algorithms to accurately interpret and understand text. Understanding idioms, sarcasm, and slang poses a challenge for NLP models, as these elements are highly context-dependent and can vary greatly across different cultures and languages. Resolving ambiguity and understanding context remains a major challenge in implementing NLP in AI.
Multilingualism
Another challenge in implementing NLP in AI is handling multiple languages. NLP models trained on one language may not perform well on text in another language, as language structures, syntax, and semantics can vary significantly between languages. Developing multilingual NLP models that can effectively process and understand text in multiple languages is a complex task that requires extensive linguistic knowledge and computational resources.
Privacy and Ethical Concerns
The use of NLP in AI raises privacy and ethical concerns related to data security and user consent. NLP models that process sensitive user data must comply with data protection regulations and privacy laws, and ensuring that user data is handled securely and ethically is essential for the responsible implementation of NLP in AI applications. Developing ethical guidelines for the use of NLP in AI is crucial to address these concerns and build trust with users.
Scalability and Performance
Scalability and performance are key challenges in implementing NLP in AI, especially when dealing with large-scale text data and real-time processing requirements. NLP models must be able to scale efficiently and handle increasingly complex tasks to meet the growing demands of AI applications. Improving the computational efficiency and speed of NLP algorithms is crucial for ensuring that NLP can be effectively integrated into AI systems.
Interpretable and Explainable Models
Interpreting and understanding the decisions made by NLP models is another challenge in implementing NLP in AI. Interpretable and explainable models are essential for building trust with users and ensuring that NLP algorithms can be scrutinized and verified for fairness and accuracy. Developing methods for interpreting and explaining the behavior of NLP models is an ongoing area of research in AI ethics and transparency.
In conclusion, implementing NLP in AI presents several challenges that need to be addressed to fully leverage the power of natural language understanding in artificial intelligence applications. By tackling these challenges and developing innovative solutions, the potential of NLP in AI can be realized to create intelligent systems that can interact with users in a more intuitive and natural way.
Future Trends in NLP and AI
As Natural Language Processing (NLP) continues to evolve, there are several exciting future trends that are expected to shape the field of Artificial Intelligence (AI). These trends hold the potential to revolutionize how humans interact with machines and how information is processed and analyzed.
Conversational AI
One of the most prominent future trends in NLP and AI is the advancement of Conversational AI. This involves creating more human-like interactions between machines and users, allowing for natural language conversations. With the rise of virtual assistants like Siri and Alexa, we can expect to see more sophisticated chatbots that are capable of understanding context and emotions, providing personalized responses, and offering more intuitive interactions.
Multimodal NLP
Another key trend in the future of NLP and AI is the development of Multimodal NLP systems. These systems will be able to process and understand various types of data inputs, such as text, images, videos, and audio. By integrating different modalities, these systems can provide more comprehensive contextual understanding, enabling a more holistic analysis of information.
Explainable AI
Explainable AI is a growing trend that focuses on making AI systems more transparent and interpretable. This is particularly important in applications where ethical considerations and accountability are crucial. In the context of NLP, explainable AI can help users understand how and why certain decisions are made by AI models, leading to greater trust and confidence in the technology.
Transfer Learning
Transfer learning is another important future trend in NLP and AI. This technique involves pretraining a model on a large dataset and then fine-tuning it on a smaller, task-specific dataset. By leveraging knowledge learned from one task to improve performance on another, transfer learning can help reduce the need for extensive training data and improve efficiency in developing NLP models.
Personalization and Customization
Personalization and customization are key trends that are expected to reshape how NLP and AI technologies are used. By tailoring AI systems to individual preferences and behaviors, companies can deliver more personalized experiences to users, leading to higher user engagement and customer satisfaction. This trend will likely drive the development of adaptive NLP algorithms that can dynamically adjust to user input and feedback.
Ethical AI
Ethical considerations are becoming increasingly important in the field of NLP and AI. As AI technologies become more pervasive in society, there is a growing need to address ethical concerns such as bias, privacy, and fairness. Future trends in NLP and AI will focus on developing ethical frameworks and guidelines to ensure that AI systems are developed and deployed in a responsible and ethical manner.
In conclusion, the future of NLP and AI holds tremendous potential for innovation and advancement. By embracing these future trends and addressing the challenges that lie ahead, we can unlock new possibilities in how we interact with technology and harness the power of AI for the betterment of society.