Exploring the Impact of Conversational AI on Natural Language Processing
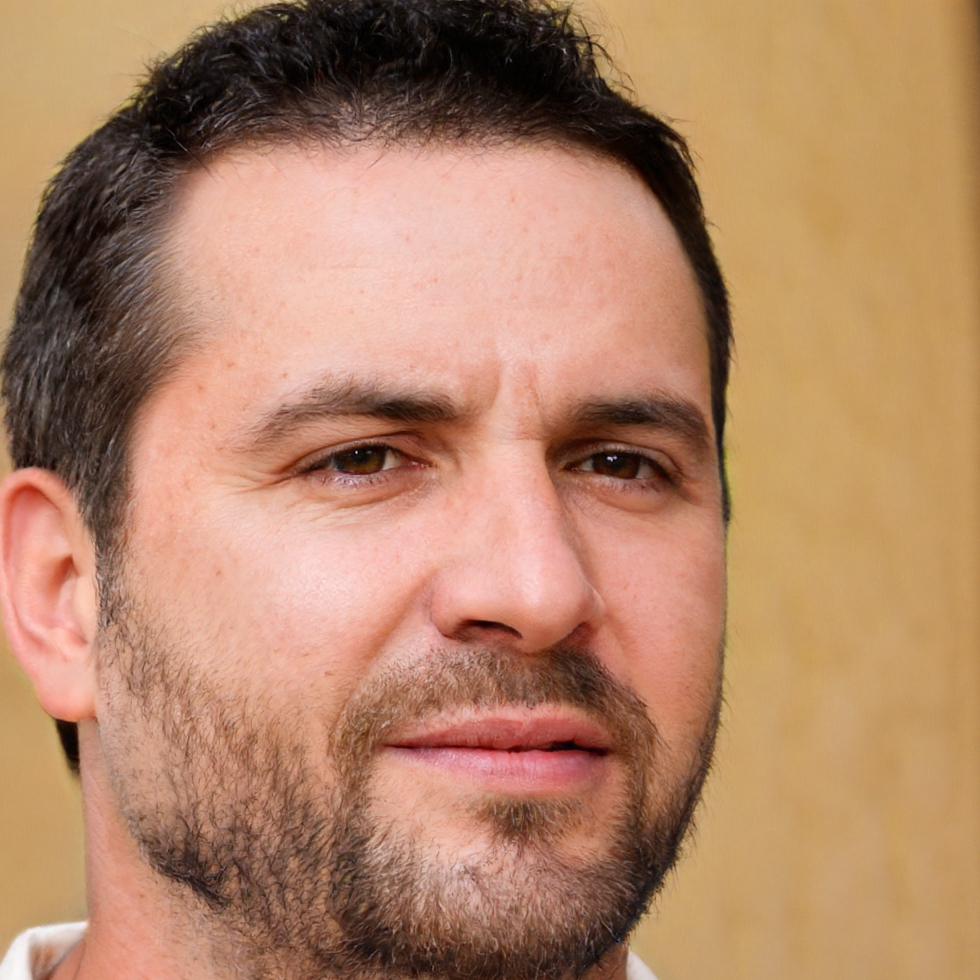
Author
Oliver Thompson"Exploring the Impact of Conversational AI on Natural Language Processing" delves into the intersection of two groundbreaking technologies - Conversational AI and Natural Language Processing (NLP). This article explores the evolution of NLP, provides an overview of Conversational AI, discusses challenges in implementing this technology, examines its applications in various industries, and looks into how Conversational AI can improve NLP. Additionally, ethical considerations in the development of Conversational AI are also highlighted.
Introduction
With the rapid advancements in technology and the increasing demand for seamless human-computer interactions, Conversational AI has emerged as a groundbreaking technology that is poised to revolutionize the way we communicate with machines. This technology, which combines elements of Artificial Intelligence (AI), Natural Language Processing (NLP), and Machine Learning (ML), enables machines to understand and respond to human language in a more natural and human-like manner.
The development of Conversational AI is driven by the growing popularity of virtual assistants and chatbots in various industries, as well as the increasing dependency on voice-controlled devices such as smart speakers and smartphones. These applications have transformed the way we interact with technology, making it more intuitive and efficient.
In this article, we will delve into the impact of Conversational AI on Natural Language Processing (NLP) and explore how this technology is shaping the future of communication. We will examine the evolution of NLP, provide an overview of Conversational AI, discuss the challenges in implementing this technology, explore its applications in various industries, and analyze how Conversational AI is improving NLP. Additionally, we will address the ethical considerations that arise in the development of Conversational AI.
Through this exploration, we aim to shed light on the potential of Conversational AI to enhance the way we interact with machines and empower businesses to provide better customer experiences. Join us on this journey as we unravel the fascinating world of Conversational AI and its impact on NLP.
Evolution of Natural Language Processing
Natural Language Processing (NLP) has a long and fascinating history that dates back to the 1950s. The initial focus of NLP was on creating programs that could analyze and understand human language. Early attempts at NLP involved rule-based systems that relied on a set of predefined rules to process and interpret text. These systems were limited in their capabilities and struggled to handle the complexity and nuance of language.
However, over the decades, advancements in machine learning and artificial intelligence have revolutionized the field of NLP. The introduction of statistical models and neural networks has enabled more sophisticated approaches to language understanding. These models are capable of learning from large amounts of text data and can generalize to new, unseen data.
One of the key milestones in the evolution of NLP was the development of the Word2Vec algorithm by Google in 2013. This algorithm was able to learn word embeddings by training on a large corpus of text, capturing semantic relationships between words. Word embeddings have since become a cornerstone of many NLP applications, enabling tasks such as sentiment analysis, named entity recognition, and machine translation.
Another significant breakthrough in NLP came with the rise of transformer models, such as BERT and GPT-3, which use self-attention mechanisms to capture long-range dependencies in text. These models have achieved state-of-the-art performance on a wide range of NLP tasks and have opened up new possibilities for natural language understanding.
The field of NLP continues to evolve rapidly, with researchers exploring new architectures, techniques, and applications. Recent trends in NLP include the integration of multimodal data, such as text and images, the development of more interpretable models, and the exploration of cross-lingual and zero-shot learning.
Overall, the evolution of NLP has been driven by a convergence of advances in machine learning, computational power, and data availability. As NLP technology continues to mature, we can expect to see even more impressive applications in areas such as healthcare, finance, and customer service.
Overview of Conversational AI
Conversational AI, also known as chatbots or virtual assistants, is a technology that enables machines to have natural language conversations with humans. Unlike traditional computer interfaces that require users to navigate through menus and options, conversational AI systems allow users to interact with machines in a more human-like manner, using natural language.
The goal of conversational AI is to enable seamless interactions between humans and machines, making it easier for users to get information, complete tasks, or solve problems. This technology utilizes a variety of natural language processing techniques to understand and respond to human language, enabling it to carry out conversations that are contextually relevant and meaningful.
Conversational AI has evolved significantly in recent years, with advancements in machine learning and artificial intelligence enabling more sophisticated and intelligent interactions. These systems can now understand natural language inputs, handle complex queries, and even learn from previous interactions to improve the quality of their responses over time.
One of the key components of conversational AI is natural language understanding (NLU), which involves processing and interpreting human language to derive meaning and intent. This enables the system to understand user queries, extract relevant information, and generate appropriate responses.
Another important aspect of conversational AI is natural language generation (NLG), which involves creating human-like responses based on the information provided by the user. NLG enables the system to generate responses that are coherent, relevant, and contextually appropriate.
Overall, conversational AI has a wide range of applications across industries, including customer service, healthcare, finance, and more. As organizations look to improve customer experiences and streamline operations, conversational AI technologies are becoming increasingly popular and essential in today's digital world.
Challenges in Implementing Conversational AI
Implementing Conversational AI poses several challenges that organizations need to address in order to successfully integrate this technology into their operations. Some of the key challenges include:
1. Natural Language Understanding
One of the primary challenges in implementing Conversational AI is achieving accurate natural language understanding. This involves the ability of the system to interpret and comprehend the context and intent behind user queries or commands. Ambiguity in language, slang, idioms, and cultural nuances can make it difficult for AI systems to accurately interpret user input.
2. Contextual Understanding
Conversational AI systems need to have a deep understanding of context in order to provide relevant and meaningful responses. However, maintaining context throughout a conversation can be challenging, especially in long and complex interactions where the user may introduce new topics or shift focus.
3. Integration with Existing Systems
Integrating Conversational AI with existing IT systems and data sources within an organization can be a complex task. Ensuring seamless data flow and integration between the AI system and other systems is crucial for efficient operation and accuracy.
4. Training Data Quality
The effectiveness of Conversational AI systems heavily relies on the quality and quantity of training data used to build and train the models. Obtaining high-quality labeled data that adequately represents the diversity of user queries and intents can be a challenge, especially in specialized domains or languages.
5. User Experience
Providing a seamless and intuitive user experience is essential for the success of Conversational AI applications. Designing natural and engaging conversations that feel human-like while maintaining efficiency and accuracy can be challenging.
6. Scalability and Performance
As more users interact with a Conversational AI system, scalability and performance become critical factors. Ensuring that the system can handle increased load, provide fast response times, and scale effectively to meet growing demands is a significant challenge.
7. Security and Privacy
Protecting user data and ensuring privacy are paramount when implementing Conversational AI systems. Safeguarding sensitive information and preventing data breaches while maintaining compliance with regulatory requirements present significant challenges.
8. Multilingual Support
Providing multilingual support for Conversational AI systems can be complex, especially when dealing with different languages, dialects, and cultural nuances. Ensuring accurate translations and interpretations across languages is a challenging task.
9. Continuous Learning and Adaptation
Conversational AI systems need to continuously learn and adapt to new user queries, trends, and contexts in order to remain relevant and accurate over time. Implementing effective learning algorithms and adaptation strategies can be a challenge.
Overall, addressing these challenges requires a comprehensive approach that involves collaboration between technologists, linguists, UX designers, and domain experts to build robust and effective Conversational AI systems.
Applications of Conversational AI in Various Industries
Conversational AI has found numerous applications across various industries, revolutionizing the way businesses interact with their customers, streamline processes, and increase efficiency. Below are some of the key industries where conversational AI is making a significant impact:
1 Retail
In the retail industry, conversational AI is being used to enhance customer service and improve the shopping experience. Virtual assistants powered by conversational AI can assist customers with product recommendations, answer queries, and even process orders. Chatbots integrated into e-commerce platforms can provide personalized recommendations based on customer preferences and purchase history.
2 Healthcare
Conversational AI is transforming the healthcare industry by improving patient engagement, streamlining administrative tasks, and providing personalized support. Virtual health assistants can schedule appointments, provide medication reminders, and offer medical advice based on symptoms. Chatbots in healthcare apps can triage patient inquiries, reducing the burden on healthcare providers and improving access to care.
3 Banking and Finance
In the banking and finance sector, conversational AI is used to enhance customer service and streamline financial processes. Chatbots integrated into banking apps can provide account information, process transactions, and offer financial advice. Virtual assistants can help customers with bill payments, budgeting, and investment recommendations, improving overall financial wellness.
4 Travel and Hospitality
Conversational AI is revolutionizing the travel and hospitality industry by providing personalized customer service and seamless booking experiences. Virtual assistants can assist with hotel reservations, flight bookings, and itinerary planning. Chatbots on travel websites can answer customer inquiries, provide destination recommendations, and offer real-time updates on travel alerts.
5 Education
In the education sector, conversational AI is being used to personalize learning experiences, provide educational support, and enhance teacher-student interactions. Virtual tutors powered by conversational AI can help students with homework, offer explanations of complex concepts, and provide interactive learning experiences. Chatbots in learning platforms can assist with course navigation, answer student queries, and provide feedback on assignments.
6 Human Resources
Conversational AI is transforming the human resources industry by automating recruitment processes, improving employee engagement, and enhancing training programs. Chatbots in HR applications can assist with onboarding new employees, provide information about company policies and benefits, and answer HR-related queries. Virtual assistants can streamline the recruitment process by screening resumes, scheduling interviews, and tracking candidate progress.
7 Telecommunications
In the telecommunications sector, conversational AI is being used to enhance customer support services, streamline billing processes, and improve network management. Chatbots integrated into telecom apps can help customers troubleshoot technical issues, provide account information, and offer billing support. Virtual assistants can also assist with network monitoring and maintenance, ensuring reliable service for customers.
These are just a few examples of the diverse applications of conversational AI across various industries. As technology continues to evolve, the potential for conversational AI to transform businesses and improve customer experiences will only continue to grow.
Improving Natural Language Processing with Conversational AI
Natural Language Processing (NLP) has seen significant advancements in recent years, thanks to the integration of Conversational AI technologies. Conversational AI refers to the use of artificial intelligence (AI), machine learning, and natural language processing to enable computers to interact with humans in a conversational way. By leveraging Conversational AI, NLP systems have become more intuitive, context-aware, and capable of understanding human language in a more natural manner.
One of the primary ways in which Conversational AI is improving NLP is through contextual understanding. Traditional NLP systems often struggle to interpret ambiguous or context-dependent language. However, Conversational AI models such as chatbots and virtual assistants are designed to understand and respond to the context of a conversation. This enables them to provide more relevant and accurate responses to user queries, leading to a more human-like interaction.
Another key aspect of Conversational AI that is enhancing NLP is personalization. By analyzing user input and behavior, Conversational AI systems can tailor their responses to suit the preferences and characteristics of individual users. This not only improves the overall user experience but also allows NLP systems to learn and adapt over time, leading to more effective communication.
Furthermore, Conversational AI is facilitating multimodal communication in NLP systems. Traditionally, NLP has primarily focused on text-based interactions. However, Conversational AI technologies such as voice recognition and image processing are enabling NLP systems to interpret and respond to a wider range of inputs, including speech, images, and videos. This not only expands the capabilities of NLP systems but also makes communication more accessible and intuitive for users.
In addition to these advancements, Conversational AI is also enhancing NLP through continuous learning. By leveraging machine learning algorithms, Conversational AI systems can analyze user feedback and interactions to improve their performance over time. This iterative learning process allows NLP systems to become more accurate, efficient, and capable of handling a wider range of tasks and scenarios.
Overall, the integration of Conversational AI technologies has significantly improved the capabilities and performance of NLP systems. By enabling contextual understanding, personalization, multimodal communication, and continuous learning, Conversational AI is shaping the future of NLP and revolutionizing the way humans interact with computers in a more natural and intuitive manner.
Ethical Considerations in Conversational AI Development
As Conversational AI continues to advance and become more prevalent in our daily lives, it is essential to address the ethical considerations that arise in its development and implementation. These considerations are crucial to ensuring that Conversational AI technologies benefit society while minimizing any potential harm.
One of the key ethical considerations in Conversational AI development is privacy. Conversational AI systems often collect and store large amounts of personal data in order to improve their performance. This raises concerns about how this data is being used, stored, and protected. Developers must implement robust privacy protections to safeguard sensitive user information and prevent unauthorized access.
Another important ethical consideration is transparency. Users interacting with Conversational AI systems should be able to understand how the system works, including how decisions are made and what data is being used. Explainability is crucial to building trust with users and ensuring that they feel comfortable using these technologies.
Bias is another ethical consideration that must be addressed in Conversational AI development. Bias can creep into AI systems through the data they are trained on, leading to unfair or discriminatory outcomes. Developers must be vigilant in identifying and mitigating bias to ensure that Conversational AI systems treat all users fairly and equitably.
Furthermore, accountability is a key ethical consideration in Conversational AI development. When something goes wrong with a Conversational AI system, it should be clear who is responsible and accountable for addressing the issue. Developers and organizations must establish clear lines of accountability to ensure that users are protected and have recourse in case of harm.
Lastly, safety is a crucial ethical consideration in Conversational AI development. Conversational AI systems have the potential to impact users' lives in significant ways, so it is essential to ensure that these systems are safe and reliable. Developers must prioritize safety testing and risk assessment to mitigate any potential harms that could arise from the use of Conversational AI technologies.
In conclusion, addressing these ethical considerations in Conversational AI development is essential to harnessing the full potential of these technologies while ensuring that they benefit society in a responsible and ethical manner. By prioritizing privacy, transparency, bias mitigation, accountability, and safety, developers can build Conversational AI systems that enhance our lives while upholding ethical standards.