Deep Learning in Finance: Applications and Opportunities in AI
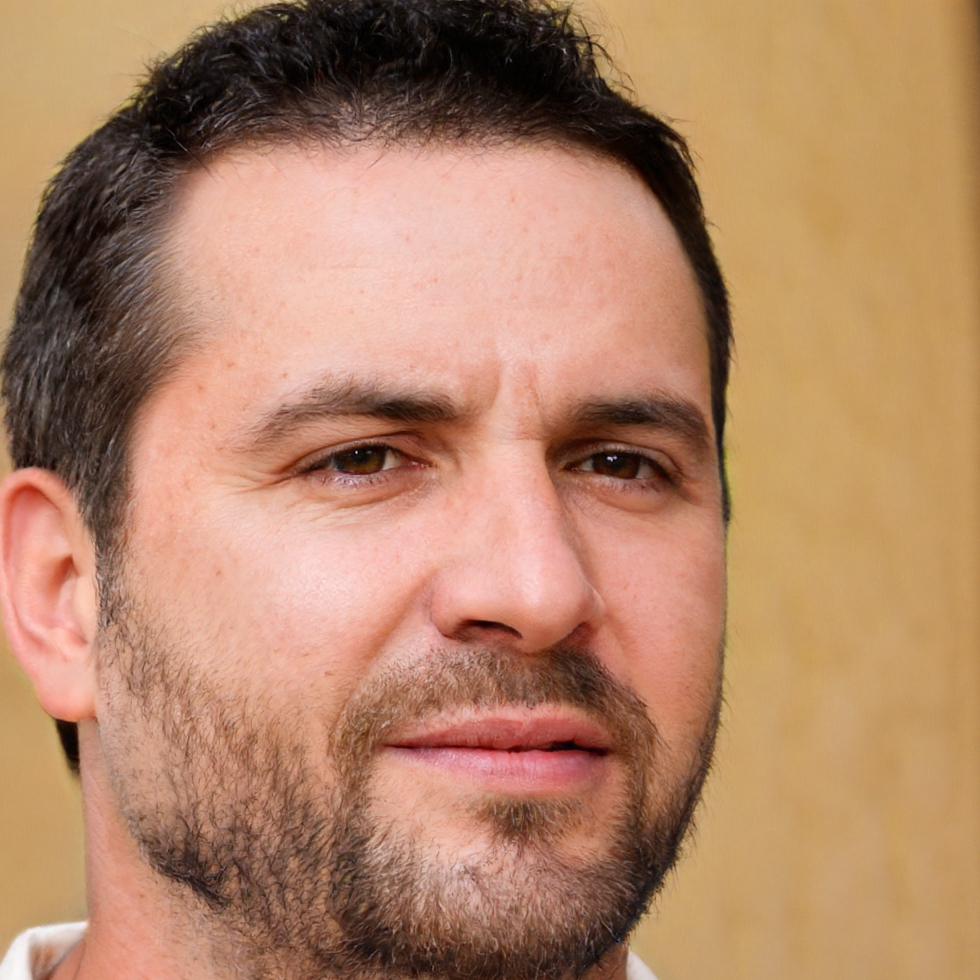
Author
Oliver ThompsonThis article provides an overview of deep learning in the field of finance and explores the current applications of this technology in financial markets. It delves into key areas such as algorithmic trading, risk management, and customer service, highlighting the impact of deep learning in these domains. Additionally, the article discusses the opportunities for deep learning in finance, including predictive analytics, personalized financial recommendations, and automated trading strategies. It also addresses the challenges and limitations in implementing deep learning in finance and presents future directions and trends in this exciting field.
Overview of Deep Learning in Finance
In recent years, deep learning has emerged as a powerful tool in various industries, including finance. Deep learning is a subset of artificial intelligence (AI) that utilizes neural networks to simulate and recognize patterns in data. Its ability to learn from large amounts of data and make predictions based on that data has made it a valuable asset for financial institutions looking to gain a competitive edge in the market.
In the context of finance, deep learning models are being used to analyze vast amounts of data such as historical stock prices, market trends, and economic indicators. These models can identify complex patterns and relationships within the data that may not be immediately apparent to human analysts. By using deep learning algorithms, financial institutions can make more informed decisions, mitigate risks, and optimize their processes.
One of the key advantages of deep learning in finance is its ability to adapt to changing market conditions. Traditional financial models are often static and rely on predetermined rules, whereas deep learning models can continuously learn and evolve as new data becomes available. This flexibility allows financial institutions to respond quickly to market shifts and make more accurate predictions about future trends.
Moreover, deep learning in finance has led to advancements in algorithmic trading, risk management, fraud detection, and customer service. These applications have not only improved the efficiency of financial processes but have also enhanced the overall customer experience.
As the financial industry continues to embrace deep learning, there are still challenges and limitations that need to be addressed. These include issues related to data quality, interpretability of results, and ethical considerations. However, with ongoing research and development, the potential for deep learning in finance is limitless. This article will explore the current applications, opportunities, challenges, and future trends of deep learning in finance.
Current Applications of Deep Learning in Financial Markets
Deep learning has revolutionized the way financial institutions operate in recent years. Its ability to analyze vast amounts of data and identify complex patterns has led to a wide range of applications in financial markets. Some of the current applications of deep learning in this field include:
2.1. Algorithmic Trading
One of the most popular applications of deep learning in financial markets is in algorithmic trading. Deep learning models can analyze historical trading data, market trends, and other factors to make real-time trading decisions. These models can adapt to changing market conditions and make predictions based on the vast amounts of data they have been trained on. This can lead to more accurate trading strategies and potentially higher profits for financial institutions.
2.2. Risk Management and Fraud Detection
Another important application of deep learning in financial markets is in risk management and fraud detection. Deep learning models can analyze a variety of factors to identify potential risks in financial transactions and detect any signs of fraudulent activity. By using deep learning algorithms, financial institutions can reduce the likelihood of fraud and minimize risk, ultimately saving money and protecting their reputation.
2.3. Customer Service and Chatbots
Deep learning is also being used in financial markets to improve customer service and communication through the use of chatbots. These AI-powered chatbots can interact with customers, answer their questions, and provide them with personalized recommendations based on their financial history and preferences. This not only improves customer satisfaction but also reduces the workload on human customer service representatives, allowing them to focus on more complex issues.
Overall, the current applications of deep learning in financial markets are diverse and impactful. From algorithmic trading to risk management and customer service, deep learning is transforming the way financial institutions operate and interact with customers. As technology continues to advance, we can expect to see even more innovative applications of deep learning in the financial sector.
Opportunities for Deep Learning in Finance
Deep learning technology has opened up a plethora of opportunities for the finance industry to revolutionize traditional practices and enhance decision-making processes. In this section, we will explore some of the key opportunities that deep learning offers in the realm of finance.
Predictive Analytics
One of the most promising applications of deep learning in finance is predictive analytics. By leveraging powerful neural networks, financial institutions can analyze vast amounts of historical data to forecast future market trends, stock prices, and customer behavior with a high degree of accuracy. This enables financial experts to make better-informed decisions, optimize investment strategies, and mitigate risks effectively.
Personalized Financial Recommendations
Deep learning algorithms excel in processing and analyzing customer data to provide personalized financial recommendations. By utilizing advanced machine learning techniques, financial institutions can tailor investment portfolios, insurance plans, and savings strategies to meet the unique needs and goals of individual clients. This not only enhances customer satisfaction and loyalty but also drives revenue growth and marketshare.
Automated Trading Strategies
Another promising opportunity for deep learning in finance is the development of automated trading strategies. By training neural networks on historical market data and real-time market conditions, financial institutions can create sophisticated algorithms that execute trades automatically based on predefined criteria. This enhances the efficiency and accuracy of trading activities, reduces human error, and maximizes returns on investment.
In conclusion, the opportunities for deep learning in finance are vast and diverse. From predictive analytics to personalized financial recommendations and automated trading strategies, deep learning technology has the potential to transform the way financial institutions operate and deliver value to their customers. By embracing this cutting-edge technology, financial institutions can stay ahead of the curve, drive innovation, and achieve sustainable growth in the dynamic and competitive finance industry.
Challenges and Limitations in Implementing Deep Learning in Finance
Deep learning has revolutionized various industries, including finance, by providing advanced machine learning capabilities. However, the implementation of deep learning models in the financial sector comes with challenges and limitations that need to be addressed for successful integration. In this section, we will discuss some of the key challenges and limitations faced when implementing deep learning in finance.
Data Quality and Quantity
One of the major challenges in implementing deep learning in finance is the availability of high-quality and sufficient data. Financial markets generate vast amounts of data in real-time, but this data is often noisy, incomplete, and subject to bias. Additionally, historical financial data may not accurately represent future market conditions, leading to model overfitting. Ensuring the quality and quantity of data used for training deep learning models is crucial for their reliability and accuracy.
Interpretability and Explainability
Another challenge in implementing deep learning in finance is the lack of interpretability and explainability of the models. Deep learning models are often considered as black boxes due to their complex neural networks and high level of abstraction. This lack of transparency makes it difficult for financial institutions to understand and trust the decisions made by the models. Interpretable and explainable deep learning models are essential for regulatory compliance, risk management, and ensuring ethical use of AI in finance.
Computational Resources and Speed
Deep learning models require significant computational resources and processing power to train and execute complex neural networks. Financial institutions may face challenges in scaling up their computational infrastructure to accommodate the needs of deep learning models. Additionally, the speed of deep learning algorithms can impact their real-time applications in high-frequency trading and risk management. Optimizing the computational efficiency of deep learning models is essential for their practical implementation in finance.
Overfitting and Generalization
Overfitting is a common challenge in deep learning, where a model learns the noise in the training data rather than the underlying patterns. In finance, overfitting can lead to misleading predictions and poor performance of deep learning models in real-world scenarios. Ensuring the generalization of deep learning models across different market conditions and data samples is crucial for their robustness and reliability. Techniques such as regularization and cross-validation can help mitigate the risk of overfitting in deep learning models.
Regulatory Compliance and Ethical Challenges
Financial institutions are subject to regulatory requirements and compliance standards that govern the use of AI and machine learning in finance. Implementing deep learning models in finance raises ethical considerations related to transparency, bias, and privacy. Ensuring compliance with regulatory frameworks and addressing ethical challenges are essential for the adoption and sustainability of deep learning in the financial sector.
Human Expertise and Collaboration
Despite the advancements in deep learning technology, human expertise and collaboration remain essential for the successful implementation of deep learning in finance. Domain knowledge and expertise are required to interpret the outputs of deep learning models, validate their predictions, and make informed decisions based on the model recommendations. Collaborations between data scientists, financial analysts, and domain experts are critical for leveraging the capabilities of deep learning in finance effectively.
In conclusion, while deep learning offers significant opportunities for innovation in the financial sector, there are challenges and limitations that need to be addressed for successful implementation. By overcoming these challenges, financial institutions can harness the power of deep learning to improve decision-making, risk management, and customer services in the rapidly evolving financial markets.
Future Directions and Trends in Deep Learning for Finance
In recent years, deep learning has made significant strides in financial markets, demonstrating its potential to transform the industry. As more data becomes available and computing power continues to increase, the possibilities for deep learning in finance are expanding rapidly. Here are some key future directions and trends that are expected to shape the application of deep learning in finance:
Enhanced Risk Management
One of the primary areas where deep learning is expected to have a major impact is in risk management. By leveraging advanced neural networks, financial institutions can improve their risk models and detect anomalies more effectively. This enhanced risk management capability will help companies better manage and mitigate financial risks, leading to a more stable and secure financial ecosystem.
Explainable AI
As the complexity of deep learning algorithms continues to increase, there is a growing need for explainable AI in finance. Explainable AI refers to models that can justify their decisions in a transparent and understandable manner. This is crucial in finance, where regulators and stakeholders require insight into model decisions to ensure compliance and trust. Expect to see a rise in explainable AI solutions tailored for the financial industry.
Ethical AI
The ethical implications of AI and deep learning are becoming increasingly important, especially in finance where decisions can have profound impacts on individuals and the economy. Ethical AI frameworks will be developed to ensure that deep learning models are used responsibly and fairly. This includes addressing bias in data, transparency in algorithmic decisions, and privacy considerations. Financial institutions will need to prioritize ethical AI practices to maintain trust with their customers and society.
Interdisciplinary Collaboration
As deep learning continues to evolve, the finance industry will see an increasing need for interdisciplinary collaboration. Experts in finance, data science, AI, and cybersecurity will collaborate to develop innovative solutions that address complex financial challenges. This collaboration will result in more robust deep learning models and practical applications that can drive value for financial institutions.
Quantum Computing
The rise of quantum computing is set to revolutionize the capabilities of deep learning in finance. Quantum computers offer unprecedented computational power that can accelerate model training, optimize investment strategies, and unlock new opportunities in financial analysis. Financial institutions investing in quantum computing technologies will gain a competitive edge in deep learning applications.
Integration of Alternative Data Sources
In the digital age, alternative data sources are proving to be valuable inputs for deep learning models in finance. Social media, satellite data, IoT devices, and other sources provide insights that can supplement traditional financial data. Integration of alternative data sources will enhance predictive capabilities, improve risk management, and generate new investment strategies. Expect to see a growing trend of alternative data integration in finance applications.
Continued Regulation and Compliance
As deep learning becomes more prevalent in finance, regulators will play a crucial role in guiding its ethical and responsible use. Compliance with regulatory requirements will be paramount for financial institutions deploying deep learning models. Expect to see ongoing evolution of regulatory frameworks to address the challenges and opportunities presented by AI in the financial sector.
Democratization of AI in Finance
The democratization of AI and deep learning tools will empower a wider range of financial professionals to leverage advanced machine learning capabilities. Low-code and no-code platforms will make AI accessible to non-technical users, enabling them to develop custom solutions for specific financial tasks. This democratization will drive innovation and efficiency in finance, leading to smarter investment decisions and improved customer experiences.
As deep learning continues to evolve and transform the finance industry, it is essential for stakeholders to stay updated on the latest developments and trends. By embracing these future directions and trends, financial institutions can leverage the power of AI to drive growth, innovation, and sustainability in the financial landscape.