Conversational AI vs. Traditional Conversational Systems: A Comparison
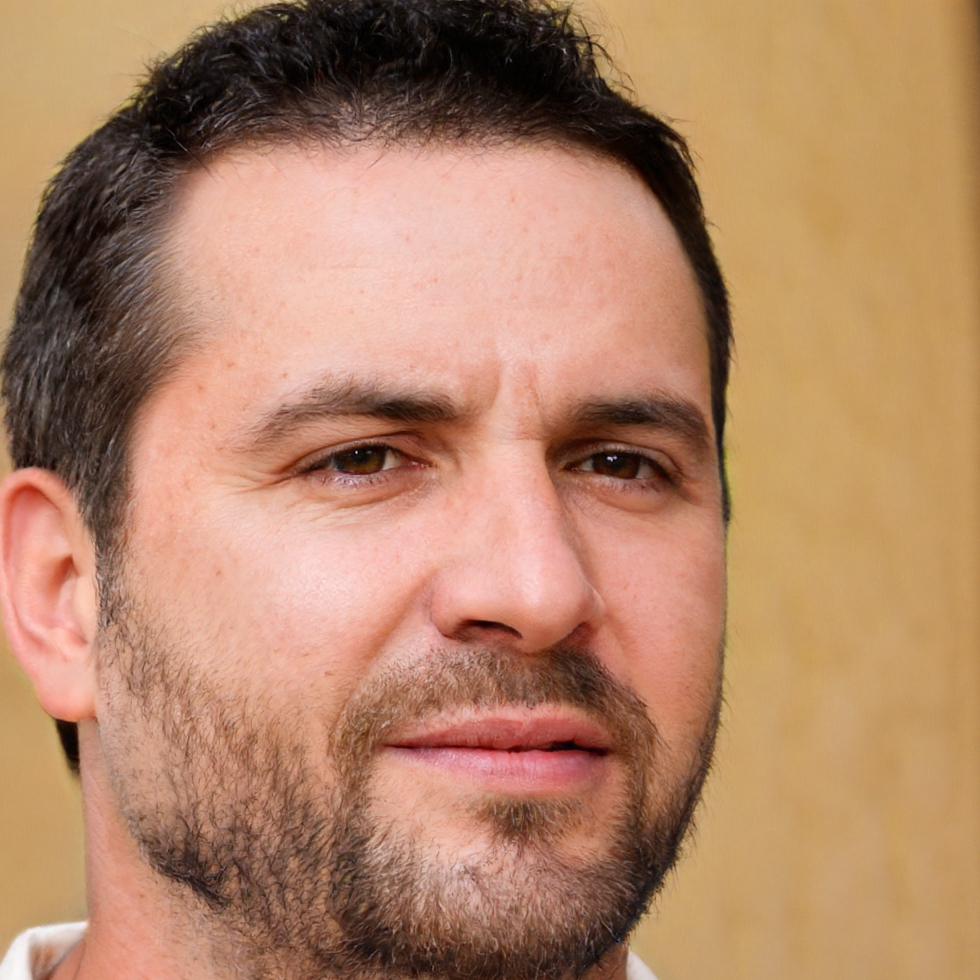
Author
Oliver ThompsonConversational AI and Traditional Conversational Systems play a significant role in shaping the future of communication. In this article, we will delve into the key differences between these two types of systems, explore their technological advancements, examine their use cases, discuss the challenges they face, and speculate on the future trends in this dynamic field.
Introduction
In recent years, Conversational AI has emerged as a disruptive technology that is transforming the way businesses interact with their customers. Conversational AI refers to AI-powered systems that can engage in natural language conversations with humans. These systems are designed to simulate human conversation and provide personalized responses to user queries.
Traditional conversational systems, on the other hand, are rule-based systems that follow a predefined set of rules to interact with users. While these systems have been around for decades and have been effective in certain use cases, they are limited in their ability to understand and respond to the nuances of human language.
The rise of Conversational AI has been driven by advances in natural language processing (NLP) and machine learning technologies. These advancements have enabled AI systems to analyze and understand human language in a more sophisticated way, leading to more accurate and contextually relevant responses.
In this article, we will explore the key differences between Conversational AI and traditional conversational systems, the technological advancements that have fueled the growth of Conversational AI, the various use cases where Conversational AI is being deployed, the challenges that organizations may face when implementing Conversational AI, and the future trends that are shaping the evolution of Conversational AI technology.
Overview
In this section, we will provide a comprehensive overview of Conversational AI and Traditional Conversational Systems, comparing their features, functionalities, and applications in various industries.
Conversational AI refers to the use of artificial intelligence technologies to create intelligent virtual agents that can engage in natural language conversations with users. These agents can understand human language, interpret intent, and provide relevant responses autonomously. Conversational AI systems are powered by machine learning algorithms and natural language processing (NLP) capabilities, enabling them to learn and improve over time.
On the other hand, Traditional Conversational Systems typically rely on rule-based approaches to process and respond to user input. These systems follow pre-defined scripts and decision trees to interact with users, limiting their ability to handle complex queries and adapt to new scenarios. Traditional Conversational Systems are often based on static rules and lack the learning capabilities of Conversational AI.
When comparing Conversational AI and Traditional Conversational Systems, it is important to consider their performance, scalability, flexibility, and user experience. Conversational AI excels in understanding natural language, context, and sentiment, leading to more personalized and engaging interactions. In contrast, Traditional Conversational Systems may struggle with ambiguity, context switching, and maintaining a coherent dialogue.
In recent years, the adoption of Conversational AI has surged across industries such as customer service, healthcare, education, and e-commerce. Organizations are leveraging Conversational AI to automate customer interactions, improve service efficiency, and deliver seamless user experiences. Traditional Conversational Systems are gradually being replaced by more advanced Conversational AI solutions that offer superior performance and adaptive capabilities.
Overall, the shift towards Conversational AI signals a new era in communication technology, enabling businesses to connect with customers in more meaningful and efficient ways. As Conversational AI continues to evolve and mature, it is poised to revolutionize how we interact with machines and access information in the digital age.
Key Differences
When comparing Conversational AI with Traditional Conversational Systems, there are several key differences that set them apart. These variances play a crucial role in determining the capabilities and effectiveness of these systems in various applications. In this section, we will explore the main variations between Conversational AI and Traditional Conversational Systems:
Natural Language Understanding:
One of the fundamental disparities between Conversational AI and Traditional Conversational Systems lies in their Natural Language Understanding capabilities. Conversational AI systems leverage advanced algorithms and machine learning techniques to interpret and process human language with a high degree of accuracy. These systems can decipher nuances, context, and intent behind user inputs, enabling them to provide more personalized and relevant responses.
In contrast, Traditional Conversational Systems typically rely on rule-based approaches and predefined scripts to understand user queries. These systems may struggle with complex language structures, slang, or variations in phrasing, leading to inaccurate or generic responses. The limitations in Natural Language Understanding can hinder the user experience and limit the effectiveness of Traditional Conversational Systems.
Contextual Understanding:
Conversational AI excels in Contextual Understanding, allowing the system to maintain context throughout a conversation and provide more coherent responses. By leveraging techniques such as contextual memory and dialogue management, Conversational AI can remember previous interactions, understand references, and adapt its responses based on the ongoing conversation. This capability enhances the conversational flow and makes interactions more engaging and effective.
On the other hand, Traditional Conversational Systems may struggle to maintain context and continuity in conversations. Without robust Contextual Understanding mechanisms, these systems may treat each query in isolation, leading to disjointed conversations and limited personalization. The lack of contextual awareness can hinder the user experience and reduce the overall effectiveness of Traditional Conversational Systems.
Personalization and Adaptability:
Conversational AI excels in Personalization and Adaptability, allowing the system to tailor responses based on user preferences, behavior, and history. By leveraging user profiling, machine learning, and personalized recommendation algorithms, Conversational AI can provide individualized responses, product recommendations, and assistance to users. This level of personalization enhances user engagement and satisfaction, leading to more meaningful interactions.
In contrast, Traditional Conversational Systems may struggle to deliver personalized experiences due to their reliance on static scripts and rules. These systems often provide generic responses that do not take into account the user's specific needs or preferences. The lack of Personalization and Adaptability in Traditional Conversational Systems can limit the utility and relevance of the interactions, resulting in lower user satisfaction and engagement.
Technological Advancements
Technological advancements have played a crucial role in the evolution of conversational AI, enhancing its capabilities and making it more sophisticated than traditional conversational systems. The following are some of the key advancements that have propelled conversational AI to the forefront of technology:
Natural Language Processing (NLP)
Natural Language Processing (NLP) is a branch of artificial intelligence that enables machines to understand, interpret, and generate human language. This technology has significantly improved the ability of conversational AI systems to comprehend and respond to human speech in a more natural and contextually relevant way.
Machine Learning
Machine learning algorithms have revolutionized conversational AI by enabling systems to learn from data and improve their performance over time. These algorithms allow conversational AI to adapt to user input, personalize responses, and continuously enhance the user experience.
Deep Learning
Deep learning is a subset of machine learning that uses neural networks to simulate human brain functions and process vast amounts of data. Deep learning has enhanced conversational AI systems' ability to understand complex patterns in speech and text and generate more human-like responses.
Sentiment Analysis
Sentiment analysis technology allows conversational AI systems to analyze the sentiment behind user input, such as emotions, attitudes, and opinions. By understanding the user's sentiment, conversational AI can tailor its responses to provide more empathetic and personalized interactions.
Multi-Modal Capabilities
Recent advancements in multi-modal capabilities have enabled conversational AI systems to process various forms of input, including text, speech, images, and videos. This allows users to interact with conversational AI in a more intuitive and natural way, expanding the possibilities for personalized and engaging conversations.
Integration with IoT Devices
Conversational AI systems are increasingly being integrated with Internet of Things (IoT) devices, enabling users to interact with their connected devices through voice commands and natural language. This integration has made conversational AI more accessible and convenient for users, opening up new possibilities for smart home automation and control.
Real-Time Processing
Technological advancements in real-time processing have improved the speed and efficiency of conversational AI systems, enabling them to deliver instantaneous responses and engage in dynamic conversations with users. Real-time processing has enhanced the user experience by reducing response times and creating more interactive and engaging interactions.
Overall, technological advancements in natural language processing, machine learning, deep learning, sentiment analysis, multi-modal capabilities, IoT integration, and real-time processing have significantly enhanced the capabilities of conversational AI systems, making them more intelligent, responsive, and user-friendly than traditional conversational systems. As these advancements continue to evolve, conversational AI is poised to revolutionize the way we interact with technology and enhance various industries' capabilities and efficiencies.
Use Cases
When it comes to Conversational AI and traditional conversational systems, both have a wide range of use cases across various industries. Here are some of the key areas where these technologies are making a significant impact:
Customer Service
Conversational AI has revolutionized the way companies interact with their customers. Chatbots powered by Conversational AI can provide instant support, answer common queries, and even handle more complex issues by escalating them to a human agent. This has led to improved customer satisfaction and reduced response times.
Healthcare
In the healthcare industry, Conversational AI is being used to streamline patient communication and support. Virtual health assistants can schedule appointments, provide medication reminders, and even offer medical advice based on symptoms reported by the patient. This technology has the potential to improve patient outcomes and reduce healthcare costs.
Retail
Conversational AI is helping retailers enhance the customer shopping experience. Chatbots can assist customers with product recommendations, answer questions about products, and even facilitate the ordering process. This personalized assistance can lead to increased sales and customer loyalty.
Education
In the education sector, Conversational AI is being used to create personalized learning experiences for students. Virtual tutors can provide additional support outside of the classroom, help students with homework, and even administer quizzes to assess comprehension. This technology is changing the way students learn and interact with educational content.
Financial Services
Banks and financial institutions are leveraging Conversational AI to improve customer service and streamline operations. Chatbots can assist customers with account inquiries, provide financial advice, and even help with transactions such as transferring funds. This technology is helping financial organizations provide a more efficient and personalized customer experience.
Human Resources
In the HR field, Conversational AI is being used to automate routine tasks and improve employee engagement. Virtual HR assistants can answer common HR-related questions, assist with the onboarding process, and even conduct employee surveys to gather feedback. This technology is freeing up HR professionals to focus on more strategic initiatives.
Overall, Conversational AI is transforming the way businesses and organizations interact with their customers and employees. With its ability to provide instant support, personalized assistance, and seamless communication, Conversational AI is becoming a valuable tool in a wide range of industries.
Challenges
Conversational AI technology has rapidly advanced in recent years, enabling more natural and engaging interactions between humans and machines. However, along with these advancements come various challenges that need to be addressed for successful implementation and deployment of conversational AI systems. Some of the key challenges include:
Natural Language Understanding
One of the primary challenges in conversational AI is natural language understanding (NLU). NLU involves processing and interpreting unstructured human language inputs, which can be ambiguous, context-dependent, and filled with slang or colloquialisms. Building NLU models that accurately understand user intents, extract relevant information, and generate appropriate responses is a complex task that requires continuous training and fine-tuning.
Context Awareness
Another challenge in conversational AI is context awareness. Conversations are dynamic and fluid, often spanning multiple turns and topics. For a conversational AI system to engage effectively, it must maintain context across interactions, remember user preferences, and adapt responses based on the evolving conversation flow. Ensuring that the system can contextually understand and respond to user inputs is crucial for delivering a personalized and seamless user experience.
Multimodal Interactions
With the rise of multimodal interfaces (e.g., voice, text, visuals), conversational AI systems are expected to support multimodal interactions seamlessly. This presents a challenge in designing and implementing AI models that can process and integrate information from various modalities, such as spoken language, text inputs, images, and videos, to provide cohesive and comprehensive responses. Handling multimodal inputs and outputs requires sophisticated AI architectures and algorithms that can effectively combine different modalities to enhance the user experience.
Scalability and Performance
Scalability and performance are critical challenges in deploying conversational AI systems at scale. As the volume of interactions and users increases, the system must be able to handle scalability to accommodate the growing demand without compromising performance. Maintaining low latency, high availability, and efficient resource utilization becomes crucial in ensuring a seamless conversational experience for users across various channels and platforms.
Data Privacy and Security
Data privacy and security are paramount challenges in conversational AI, especially when handling sensitive personal or confidential information. Conversational AI systems often collect and store user data to improve personalization and user experience. However, ensuring data privacy compliance, secure data storage, and robust encryption mechanisms to protect user information from unauthorized access or breaches is essential. Implementing stringent security measures and complying with data protection regulations are vital to building trust and maintaining user confidence in conversational AI systems.
In conclusion, addressing these challenges requires a holistic approach that encompasses technological innovations, user-centered design, ethical considerations, and continuous learning. By overcoming these hurdles, conversational AI systems can deliver more intelligent, intuitive, and personalized interactions that enhance user engagement and satisfaction.
Future Trends
As technology continues to evolve at a rapid pace, the future of conversational AI and traditional conversational systems is expected to see some significant advancements and changes. Several key trends are likely to shape the direction of these technologies in the coming years.
1. Integration with IoT
One of the major trends in the future of conversational systems is the integration with the Internet of Things (IoT). With the rise of smart devices and connected homes, conversational AI and traditional systems will play a crucial role in facilitating communication between humans and machines. This integration will enable users to control their devices and access information through voice commands, making interactions more seamless and intuitive.
2. Personalization and Context Awareness
Another important trend in the future of conversational systems is the focus on personalization and context awareness. Conversational AI will become smarter and more personalized, understanding user preferences and tailoring responses to individual needs. By leveraging machine learning and natural language processing techniques, these systems will be able to provide more relevant and timely information, enhancing the overall user experience.
3. Multi-Modal Interfaces
With the increasing popularity of virtual assistants and smart speakers, the future of conversational systems will also see a shift towards multi-modal interfaces. These interfaces will combine voice with visual elements such as text, images, and videos, allowing users to interact with the system in a more natural and intuitive way. This trend will enhance the user experience and open up new possibilities for interactive communication.
4. Enhanced Security and Privacy
As conversational systems become more prevalent in various aspects of our lives, ensuring security and privacy will be a key focus in the future. Conversational AI developers and providers will need to implement robust security measures to protect user data and prevent unauthorized access. Privacy regulations such as the GDPR will also play a significant role in shaping the future of these technologies.
5. Industry-Specific Applications
In the future, we can expect to see an increase in the adoption of conversational systems in industry-specific applications. From healthcare to banking to customer service, these technologies will be used to streamline processes, improve efficiency, and enhance customer interactions. Companies will continue to invest in conversational AI solutions to gain a competitive edge in their respective markets.
6. Continued Innovation and Advancements
Overall, the future of conversational AI and traditional conversational systems will be characterized by continued innovation and advancements. As technology continues to evolve, these systems will become more sophisticated and intelligent, offering new capabilities and features that were previously unimaginable. With AI at the forefront of these developments, the possibilities are endless, and the future of conversational systems holds great promise for improving the way we interact with technology.